Chapter 5 From registration to re-identification: Exploring the interplay of data matching software in routine identification practices
Abstract
In migration management and border control, identifying individuals across data infrastructures frequently demands intricate processes involving integrating and aligning diverse data across various organizations, temporal contexts, and geographical boundaries. While existing literature predominantly focuses on first registration and identification, this chapter takes a novel empirical route by investigating the “re-identification” of applicants in bureaucratic processes within the Netherlands’ Immigration and Naturalization Service (IND). Re-identification is conceptualized as the continuous utilization and interconnection of data from various sources to ascertain whether multiple sets of identity data correspond to a singular real-world individual. Through the lens of re-identification, the research examines the iterative processes of identifying applicants across various stages of bureaucratic processes, drawing from fieldwork and interviews conducted at the IND and its data matching software provider. The study delves into the IND’s designed infrastructure for applicant re-identification, particularly the tools for searching and matching identity data. By contrasting design and practical use, the research uncovers multiple forms of data friction that may hinder re-identification. Furthermore, exploring the costs stemming from failed re-identification, manifested through duplicate records and the labor-intensive deduplication process, highlights the evolving bureaucratic re-identification practices and their links with transnational security infrastructures. The findings contribute to debates about the materiality and performativity of identification in two ways. Firstly, they redirect attention from first registration and identification to encompass re-identification practices across data infrastructures. Through an interpretative framework developed in the analysis, re-identification is further demonstrated not as a singular process but as a range of iterative practices. Secondly, the findings underscore that while integrating data matching tools for re-identification alleviates data friction, it inadvertently also comes with certain costs. This integration involves a redistribution of re-identification competencies and labor between the IND and the commercialized data matching engine and potentially shifting the burden of costs related to failed re-identification to different parts of the bureaucratic system.
- Contribution to research objectives
- To examine the relationship between identity data matching technologies and routine identification practices.
- This chapter provides an in-depth analysis of the interconnections between identity data matching technologies and routine identification practices, focusing on the operational intricacies of re-identification within migration management, such as in the context of residency or naturalization applications. Through empirical investigation, the chapter explores the utilization and interconnection of data from various sources to ascertain whether multiple database records correspond to individual applicants. By comparing the design of data matching tools in contrast to their real-world implementation, the comparison unveils multiple forms of data friction that can impede the IND’s re-identification processes. One form of friction arises from variations in the precision and accuracy of identity data during its transformation across different mediums, which subsequently influences the formulation of search queries. Furthermore, data friction can arise from the opaque calculation of match results by the tools, making it challenging for IND staff to understand search results, leading to the need for refining search parameters and strategies. The investigation highlights the possible costs of these forms of friction by examining the consequences of unsuccessful re-identification, exemplified by duplicate records.
- Infrastructural inversion strategy used
- Second Inversion Strategy — Data Practices
- This chapter uses the second infrastructural inversion strategy to examine the practices related to identity matching and linking across data infrastructures. Using this strategy, the chapter’s findings highlight a significant diversity in re-identification practices within the IND. This diversity emerges in two main aspects. On the one hand, re-identification practices are characterized by the information available to staff during the process. On the other hand, the precision criteria necessary for successful re-identification exhibit significant variation. Building on these observations, the chapter develops an interpretative framework that categorizes re-identification practices based on the demands of interpreting search inputs and results. The resulting matrix recognizes re-identification not as a solitary process but as a range of iterative practices. These practices encompass a variety of scenarios, including direct applicant interactions, staff managing phone conversations, handling application forms sent via postal services, and automated re-identification processes.
- Contribution to research questions
- RQ2: How do organizations that collect information about people-on-the-move search and match for identity data in their systems? How is data about people-on-the-move matched and linked across different agencies and organizations?
- This chapter addresses RQ2 by analyzing the various re-identification practices that encompass a range of scenarios reflecting diverse re-identification practices within the IND, the broader collaborative framework of the Netherlands’ migration chain, and even extending to transnational data infrastructures. Internally within the IND, these scenarios encapsulate various contexts, from direct applicant interactions to staff managing telephone conversations and processing physical application forms. Across the broader migration chain, the utilization of the v-number identification numbers emerges as one element to match and link data across systems. However, the study also uncovers instances where re-identification efforts falter due to, for instance, inconsistencies in identification practices across the migration chain partners. Furthermore, the analysis of the deduplication process unveiled a connection to transnational systems, as the IND and migration chain partners harness data from prominent European Union information systems to forge connections among seemingly disparate records within their respective databases.
Figure 5.1: The axes pertaining to the methodological framework in relation to chapter 5.
- Contribution to the main research question
- How are practices and technologies for matching identity data in migration management and border control shaping and shaped by transnational commercialized security infrastructures?
- This chapter addresses the main research question by investigating the interplay between the re-identification practices of the IND and a commercially developed data matching system. This integration of proprietary tools, designed by a private entity, for data matching not only signifies a transformation in the IND’s re-identification approach but also underscores the redistribution of re-identification expertise and competencies as these are embedded within a proprietary data matching system. Furthermore, the chapter highlights a link between the deduplication process and transnational systems. The IND and migration chain partners effectively employ data from prominent European Union information systems, forging connections among apparently disparate records within their databases. Moreover, the findings demonstrated challenges faced by vendors in designing custom-made solutions versus standardized approaches for deduplication. The complexities of defining identity and duplicate records, inherently linked to the specific organizational context, became apparent during an upgrade of automated duplicate detection tools. These findings emphasize that re-identification processes and associated technologies are far from isolated; they are intricately entwined within broader commercialized security infrastructures.
5.1 Introduction
The stakes in identification can be high, and authorities’ use of specialized technologies to search and match identity data can significantly mediate uncertain identification outcomes. An often invoked real-life example of the complexities of identification is when one of the “Boston bombers,” Kyrgyz-American Тамeрла́н Царна́ев, was not pulled aside for questioning when leaving from and returning to JFK Airport in New York for a trip to Dagestan in the Northern Caucasus in 2012 (an area considered as a high-risk travel destination by the US government).30 In April 2013, he and his brother carried out a terrorist attack during the annual Boston Marathon. According to an investigative report for the United States House Committee on Homeland Security, which media outlets reviewed, he was mistakenly not identified as a person of interest nor questioned (Schmitt and Schmidt 2013; Winter 2014). In 2011, Russian authorities had already informed their American counterparts of his ties to terrorist organizations. Following this information exchange, the US government added him to various watch lists and databases, including the Terrorist Identities Datamart Environment, which contains information on over 1.5 million people who are either known or suspected to be international terrorists. Such watchlisting systems automatically compare data about individuals, alerting and instructing authorities on what to do when they encounter someone whose data matches a watchlist entry. Due to missing information regarding Mr Царна́ев’s date of birth and variations in the transliteration of his name, “Tsarnaev” — “Tsarnayev,” the system did not raise an alert in his case.
The case of the Boston bomber exemplifies at least three essential features of modern identification practices mediated by digital technologies: inherent difficulties, technological solutionism and flexibility in the application of regulation. First, at all levels of bureaucracy, from street-level bureaucrats to system-level bureaucracies, there are inherent difficulties in accurately identifying and confirming identities. Most organizations must cope with databases containing incomplete, not current, incorrect data — or even duplicate entries referring to the same real-world persons (Keulen 2012). Such difficulties undermine the possibility of building trust. For example, the European Union Agency for Fundamental Rights (FRA)’s investigation on the implications of data quality in EU information systems for migration and border control on fundamental rights (2018) has reported that “authorities often suspect identity fraud when cases of data quality are the real reason for concern” (p. 81). Hence, ensuring that data are correct, complete, and accurate and that they can be shared, used, and processed by different parties and information systems is deemed vital for the functioning of (bureaucratic) procedures.31
Within the context of identifying individuals in migration management and border control scenarios, inherent difficulties can be explained by the need to interconnect and harmonize diverse datasets spread across different organizations, timeframes, and geographical boundaries. For alphanumeric personal data (such as surname, date of birth, and nationality), these data quality issues can have various, often unspectacular, reasons. The case of Тамерла́н Царна́ев touches on the fact that watchlist databases need Latin characters’ names, yet, transliteration of a name can take many forms. Hence, working with different data sources usually brings challenges related to what I will term “re-identification.” With this term, I intend to encompass a spectrum of iterative identification processes where data, whether sourced from within or across organizations and collected across diverse temporal and spatial contexts, are employed and interconnected to determine if multiple database records correspond to a single real-world individual.32 Instances of such re-identification encompass diverse scenarios, ranging from cross-referencing an individual’s passport details to access their visa records, correlating flight information to identify matches on watchlists, or linking migration and law enforcement databases to unveil potential suspect identities.
Second, new technologies keep being introduced in an attempt to solve “data friction” (Edwards 2010), and re-identification should be perceived not only as being disrupted by data friction but also as a means through which technology can introduce friction. Research should consider how technologies for searching and matching identity data reconfigure re-identification practices. This point draws on materialist and performativity debate on identification (e.g., Fors-Owczynik and van der Ploeg 2015; Leese 2022; Pelizza 2021; van der Ploeg 1999; Pollozek and Passoth 2019; Skinner 2018). Following these debates, identification should not be understood as a problem of truthful representation between people and their identity data but of how data infrastructures for identity management and identification “enact” individuals as migrants, criminals, risky travelers. From this perspective, we can rethink the above quote from the Fundamental Rights Agency. Instead of asking if doubts about someone’s identity arise from inaccurate data or mistrustful border control practices, we must also consider how re-identification enacts subjects as potential identity frauds. For example, a case of potential identity fraud could be discovered by automatically matching similar biographical data. Such a materialist and performative approach can replace discussions of identity as faithful representation with accounts about how re-identification introduces novel forms of suspicion. So far, however, literature has focused on the materiality and performativity of first (and often biometric) identification and registration (e.g., biometric refugee registration), and there needs to be more discussion about how people are re-identified and enacted throughout bureaucratic practices and data infrastructures.
Third, the literature on street-level bureaucracy emphasizes that public employees put government policies into practice through their regular interactions with citizens to deal with complex situations that do not always fit neatly into the rules and regulations made by legislators (e.g., Lipsky 2010). Re-identification can be ambiguous, and there is often a lack of clear guidelines; as a result, there is considerable room for discretion. However, the problem of re-identifying applicants during bureaucratic procedures has received little attention in the literature to date. A helpful example of an identification encounter where the tension between systems, policies, and local circumstances is apparent is provided by Pelizza (2021). She describes the back-and-forth between an applicant, a police officer, and a translator to convert the applicant’s name from Arabic to Latin characters during first registration at a Greek border. The name that emerges from this identification encounter is, in Pelizza’s words, the result of a “chain of translations” of the migrant’s name from oral to written to finally end up in the information system to serve as the official version to be used to re-identify this person in future administrative procedures. The process Pelizza described is very different from an example I encountered in The Netherlands. In this case, when there are doubts or refusal to give a name, a person will be assigned a label that serves as a name and includes details about the applicant’s sex as well as the time and place of registration (e.g., “NN regioncode sex yymmdd hhmm”). In both examples, public servants re-identify people by tailoring their actions to the individual involved, all within the constraints and affordances of a given sociotechnical setting.
However, while the street-level bureaucracy literature has long debated the constraining or enabling effects of new technologies, such as those related to automated decision-making (Bovens and Zouridis 2002; Buffat 2015), it has been less specific about the entangled technologies. Nonetheless, it is clear that the expectations and materialities of data shape identification encounters. The designs and data models of technical solutions, such as those used to search for a person’s record or to determine whether two identity data records refer to the same person, embed many assumptions about those data (see also Pelizza and Van Rossem 2023; Van Rossem and Pelizza 2022), which shape bureaucratic re-identification. In the case of identity data, such tools assemble knowledge and enact equivalences between otherwise disparate naming practices. For example, the male and female family name forms might each have a slightly different final syllable, but they could still be considered equivalent. By examining how applicants of bureaucratic procedures are re-identified, this chapter intends to answer RQ2:
How do organizations that collect information about people-on-the-move search and match for identity data in their systems? How is data about people-on-the-move matched and linked across different agencies and organizations?
The research seeks to answer these questions by empirically studying re-identification at the migration and naturalization service in The Netherlands (IND). The analysis draws on data gathered through fieldwork — interviews, documents, field notes — at the data matching software supplier and the IND agency itself. The research hypothesized that the design of search and matching tools incorporates assumptions about databases and their data records, which influence and are influenced by bureaucratic re-identification practices. Assumptions like these include the possibility of incompatible naming practices and conventions, meaning databases could never be entirely accurate. Therefore, I formulated the hypothesis that utilizing data matching software could result in a redistribution of responsibilities and capabilities driven by the inherent affordances and limitations of the software itself. Specifically, I anticipated that government agents would rely less on their identification expertise and instead rely more on automated matching algorithms to retrieve identity information.
The chapter aims to contribute to the literature on the materiality and performativity of identification, particularly within the intersection of science and technology studies (STS) and critical migration, security, and border studies. The findings of this chapter can contribute to these scholarships in two ways. Firstly, while prior investigations have predominantly concentrated on initial registration, often involving biometric data, this chapter’s findings illuminate the often-overlooked processes of re-identification that transpire throughout bureaucratic procedures. The chapter sheds light on lesser-known practices of dealing with uncertain alphanumeric biographic data in migration management. Secondly, by examining routine re-identification interactions embedded within specific sociotechnical contexts, the findings demonstrate how incorporating data matching tools, intending to curb data friction, sometimes shifts the costs associated with managing ambiguous data to other actors or entities within bureaucratic systems.
The next section of this chapter will commence with a review of the background and related work that has been instrumental in conceptualizing re-identification as a bureaucratic practice, emphasizing its intersections with the materiality and performativity of identification processes. Following this, an overview of the case and methodology adopted for examining matching systems and applicant re-identification at the Netherlands’ Immigration and Naturalization Service (IND) will be presented. The chapter’s empirical case and findings will be presented across three sections. Two empirical sections will juxtapose the designed applicant identification infrastructure and its practical implementation at the IND, recognizing three forms of data friction that can impede the re-identification process. The third empirical section will delve into the costs of unsuccessful re-identification, focusing on the problems related to duplicate records and the labor-intensive deduplication process. Finally, the chapter will answer the chapter’s research question by synthesizing the diverse re-identification practices encountered throughout the empirical sections into an interpretative framework, highlighting a range of re-identification scenarios.
5.2 Conceptualizing re-identification: Bureaucratic contexts and the dynamics of identity data
Many interactions between migrants and public authorities involve forms of identification to establish or verify applicants’ identity in different steps of bureaucratic processes relating to granting asylum, issuing residency permits, naturalization, and so forth. As the literature on street-level bureaucracy has shown, public-service workers in charge do not just carry out relevant policies; they are also actively involved in interpretative work through the discretion workers use (e.g., Lipsky 2010; Collins 2016). What does it mean to regard re-identification practices as part of routine bureaucratic procedures?
5.2.1 Re-identification as a bureaucratic practice
Michael Lipsky’s book “Street-level bureaucracy: dilemmas of the individual in public services” (published in 1980) is widely credited with popularizing the concepts of street-level bureaucracy and discretion. According to this widely held view, diverse frontline public service workers influence public policy through regular interactions with the general public. As Lipsky (2010) states: “street-level bureaucrats have considerable discretion in determining the nature, amount, and quality of benefits and sanctions provided by their agencies” (13). For instance, a border patrol agent may have discretionary authority to grant entry to a traveler based on the results of an identification encounter with the traveler at passport control. Lipsky’s original argument, however, required updating in light of the increased use of digital technologies in government and the rise of e-government. Essentially, the digitalization of government agencies has compelled scholars to reconsider the role of street-level bureaucrats and their daily interactions (Bovens and Zouridis 2002; Buffat 2015; Busch and Henriksen 2018; Snellen 2002). With digitization, discretionary power is sensibly reduced as decisions are delegated to automated systems. This argument has implications for identification, which can occur not only in direct interactions between applicants and bureaucratic officers but also increasingly in automated processes mediated by digital tools.
The transformations brought about by information and communication technologies were conceptualized by Bovens and Zouridis (2002) in an influential article as occurring first at the “screen-level” and then at the “system-level” of bureaucracy. “Screen-level bureaucracies” refers to how interactions between officials and citizens have become increasingly mediated through computer screens. For instance, the personal data of a residency permit applicant is filled out using electronic template forms in a case management system. Alternatively, increasingly applicants themselves are also provided access to government information systems (Landsbergen 2004). Meanwhile, decision trees, business rules, and algorithms that model the policies and regulations will guide the decision to grant the permit in this example. “System-level bureaucracies” refers to an even higher level of automation and digitization when collecting data and carrying out routine tasks. The following is the author’s idealized description of the practitioners’ new roles in such an organization:
The members of the organization are no longer involved in handling individual cases, but direct their focus toward system development and maintenance, toward optimizing information processes, and toward creating links between systems in various organizations. Contacts with customers are important, but these almost all concern assistance and information provided by help desk staff. After all, the transactions have all been fully automated. (Bovens and Zouridis 2002, 178–79)
Within the bureaucratic processes, individuals applying for asylum may often find themselves subject to iterative identification procedures that traverse the realms of street-level, screen-level, and system-level bureaucracies. It typically commences at the street and screen levels, where front-line bureaucrats serve as the initial point of contact. These bureaucrats collect and input applicant information, funneling it into the complex interfaces of the bureaucratic systems. Subsequently, further decisions regarding data management, including updates, linkages, and corrections to applicant information, may be made at the system-level. This iterative process through bureaucratic layers underscores the importance of consistent and precise identification practices across all levels.
The concept of “re-identification” highlights the entanglement of street-level procedures and the crucial role in automated systems within system-level bureaucracies responsible for processing applications from individuals seeking services or assistance. Automated processes must correctly re-identify the distinct applicants of bureaucratic processes to make the right decisions. System development will thus be required to automatically re-identify individual cases and to ensure that data are accurate and up to date, that no duplicate entries exist, and so on. Moreover, as applicants themselves are provided access to government information systems, such as through the filling out of digital application forms, they can be assumed to become more involved in the re-identification process. This change brings an additional layer of complexity, as the accuracy and consistency of the data provided by the applicants also contribute to the success of re-identification processes. In a system-level bureaucracy, re-identification will be linked to verifying and connecting individual records across systems and organizations. [maybe this is the place to defi ne re-identification]
In the literature about the entanglement of street-level, screen-level, and system-level bureaucracies, the desirability of automation for fairness and efficiency is usually weighed against its potential negative impact on human judgement and autonomy. Buffat (2015) categorizes these debates into the “curtailment thesis” and “enablement thesis.” The former argues that information and communication technology (ICT) limits frontline officers’ discretion, transferring it to other actors. The “enablement thesis,” on the other hand, suggests that technologies play a more nuanced role by shaping interactions between technologies, workers, and citizens. A more recent perspective, the “digital discretion” literature, proposes the use of “computerized routines and analyses to influence or replace human judgement” (Busch and Henriksen 2018, 4) to adhere to policies and ensure fair and consistent outcomes. This chapter takes a further distinct STS-influenced approach, emphasizing how re-identification is intertwined with technology’s affordances and constraints that shape bureaucratic realities. Such an STS lens prompts us to be specific about the use of technologies, such as how the design of data matching systems, their embedded algorithms, and their interfaces affect the daily routines of those involved in re-identification processes and ultimately shape the re-identification outcomes.
The literature suggests that when analyzing the interplay between re-identification, discretion, and varying levels of bureaucracy, two key elements should be taken into account. Firstly, the literature suggests that identification policies are executed by public workers in their daily routines, often influenced by their discretionary powers. Secondly, it emphasizes the need to view routine identification practices within bureaucratic frameworks in the context of broader changes in their sociotechnical systems. The concept of re-identification can help make sense of the interactions between bureaucratic organizations and applicants when these interactions combine street-level interactions, screen-level processes, and system-level bureaucracies. When there are uncertainties regarding precise identification, the discretionary components of procedures can become more important. In such scenarios, the interplay between human judgement and automated mechanisms could enhance or impede re-identification. It also raises questions about potential challenges associated with unsuccessful re-identification attempts, including subsequent consequences and necessary corrective measures.
Re-identification, as introduced here, is a concept that can offer insight into the entanglement between street-level, screen-level, and system-level bureaucracies. In the realm of bureaucratic processes, data and information frequently traverse these distinct levels, presenting both challenges and opportunities for the handling of applicant data. This concept of re-identification aims to untangle the complexities that arise when individuals engage with government systems and personnel, necessitating multiple rounds of identification and verification across bureaucratic contexts. By emphasizing the iterative character of identification, re-identification highlights the recurrent need for verifying individuals’ identities. Furthermore, it underscores the pivotal role of technology, interfaces, and organizational structures in shaping identification processes within bureaucratic systems.
5.2.2 Materiality and performativity of re-identification
A different body of literature further recognizes the importance of identification as intermingled with the government’s obligations and rights (e.g., citizenship, residency), as well as coercive measures (About, Brown, and Lonergan 2013a; Caplan and Torpey 2001). As recalled in Chapter 2, scholars have conventionally placed a significant emphasis on the interconnection between the formation of modern nation-states and the development of registration and identification systems, such as the creation of civil registers or passport documents (Breckenridge and Szreter 2012; Caplan and Torpey 2001; Torpey 2018). An often preferred term for the state’s capacity to identify its citizens is the notion of legibility of Scott (1998). Scott noted how the increased interaction of states and their population (e.g., for purposes of taxation) went hand in hand with projects of standardization and legibility as attempts to identify its people unambiguously. So, in the example by Scott, while cultural naming practices are very diverse and can serve local purposes, the standardization of surnames “was a first and crucial step toward making individual citizens officially legible” (p. 71). In these practices, the identity of the person is not a problem of representation between a person and information captured about them but one of reducing multiplicity while mutually enacting subjects, states, and institutions (Lyon 2009; Pelizza 2021). What needs to be clarified is how such concept of legibility and reducing multiplicity also intersects with the notion of re-identification, as the state’s ongoing endeavor to ensure legibility involves not only initial identification but also successive processes of verifying and connecting data over time and across various contexts.
The growing body of literature at the intersection between STS and Critical Security Studies has added an important dimension to the discussion on identification by accounting for the materiality and performativity of devices and practices (Cole 2001; Gargiulo 2017; Skinner 2018; Pelizza 2021; Suchman, Follis, and Weber 2017). Bellanova and Glouftsios (2022), for instance, have studied the actors and practices involved in maintaining the EU Schengen Information System (SIS). The SIS system allows authorities to create and consult alerts on, among others, missing persons and on persons related to criminal offences. By looking at how these alerts “acquire the status of allegedly credible and accurate information that becomes available to end-users through the SIS II” (p. 2) they make evident its role in conditioning international mobility. Fors-Owczynik and van der Ploeg (2015) have shown how three systems in the Netherlands translate and frame risk categories to identify potentially risky migrants and travelers. Building on this literature, re-identification can be understood as intricately connected with the materiality and performativity of devices, shaping the evaluation of data, individuals, and organizations as accurate and trustworthy. Drawing on findings from the politics of mobility literature (Cresswell 2010; Pallitro and Heyman 2008; Salter 2013), such as observations regarding the expedited processing of certain passenger classes through trusted traveler programs at airports, these disparities can lead to divergent outcomes. In cases where discrepancies arise, individuals may be subject to heightened scrutiny and additional security measures. Conversely, consistent information across systems has the potential to expedite their passage through border controls.
Surprisingly, despite the significance of re-identification in contemporary bureaucratic practices, there remains a noticeable gap in our understanding of how practitioners navigate the complexities arising from ambiguities in personal identity data during re-identification. A case in point is highlighted in a report by the European Court of Auditors, which outlines that “when border guards check a name in SIS II [the Schengen Information System], they may receive hundreds of results (mostly false positives), which they are legally required to check manually” (ECA 2020, 31). This operational challenge, rooted in the technology’s approach to computing and presenting matching data, exemplifies how the concept of re-identification intersects with the practical realities of border control. The abundance of false positives generated by the system raises questions about how re-identification encounters are negotiated when dealing with such ambiguities and how technologies might influence these interactions.
5.2.3 Conceptualizing data friction in re-identification
Critical data studies have made it clear that data are never “raw” (Gitelman 2013) and “contain traces of their own local production” (Loukissas 2019, 67), and that work is therefore needed to put data to use. For example, a European Court of Auditors report mentions that a prominent EU information system supporting border control contains millions of potential data quality issues, such as first names recorded as surnames or missing dates of birth (ECA 2020). Many such discrepancies are likely related to work practices and issues of fitting local circumstances to global standards (Bowker and Star 1999). As Loukissas (2019) remarks, databases might contain various errors and “local knowledge [is needed] to see that such errors are not random” (p. 67). In this sense, data serve as evidence of the local conditions of their production, which, for future re-identification processes, must be linked across space and time. If data quality problems and uncertainty are facts of life (Keulen 2012), then bureaucratic organizations must cope with this uncertainty in re-identification practices.
As highlighted in Chapter 1, multiple technical mechanisms exist for dealing with such uncertainties, such as determining whether two or more data records pertain to the same real-world individual (Batini and Scannapieco 2016). Data matching techniques will compare attributes of data records and use classification methods to determine matches (Christen 2012). There are numerous classification techniques to determine matches: some are based on adhering to specific rules, while others take a more probabilistic approach. Metrics can, for example, calculate the similarity of two sequences of characters based on the number of operations required to transform one into the other. In this way, the names “Sam” and “Pam” may be considered closely related (for instance, was it a typo?). Other approaches may even calculate such similarities by comparing how names are pronounced (in English). When matching personal data, rules-based matching may include ignoring honorifics and titles (e.g., Mr., Ms., Dr.). Although these technical mechanisms for data matching are widely recognized, their practical implications in the processes of re-identification remain less evident.
The insights gleaned from Critical Data Studies indicate that investigating technical data matching mechanisms not only reveals local conditions of data productions and operational dilemmas but also offers valuable insights into re-identification. This is exemplified by the specific case of data matching aimed at discovering and resolving duplicate data records. For instance, a migrant might inadvertently be registered multiple times in a database due to technical glitches. Typically, a deduplication process (for example, as detailed in Batini and Scannapieco 2016) periodically compares each record with all others in the database to identify records pertaining to the same individual. A domain expert usually intervenes to make decisions regarding whether these matches do indeed pertain to the same individual to consolidate the multiple data into a single one.
Following Loukissas (2019), the process of “normalizing” duplicates can be “a key to learning about the heterogeneity of data infrastructures” (p. 60). Loukissas gives the example of software that identifies digital copies of books, newspapers, and objects in a digital library collection. He challenges the software’s intention to eliminate these copies, suggesting that delving into the duplicates’ origins could be more instructive. This discussion on duplicates holds relevance for re-identification in two ways. Firstly, deduplication can offer similar insights into multiple re-identification practices. Secondly, the presence of duplicates prompts another question: what are the implications for applicants and organizations of data matching failures and unsuccessful re-identification?
The complexities arising from impediments in the seamless flow of identity data may indeed be at the heart of unsuccessful data matching and re-identification processes, which can be aptly conceptualized as manifestations of “data friction” (Edwards 2010). Data friction, according to Edwards (p. 84), “refers to the costs in time, energy, and attention required simply to collect, check, store, move, receive, and access data.” Data friction signifies the barriers that disrupt the smooth flow of data across different actors, organizations, and material forms. As noted by Bates (2017), data friction is “influenced by a variety of infrastructural, sociocultural and regulatory factors interrelated with the broader political economic context,” all of which influence the movement or hindrance of data. Pelizza (2016b) explains the process of addressing data friction as a dynamic interplay between aligning and replacing infrastructural elements that facilitate data movement, where changes in one aspect impact the other. In her study of the Dutch land registry, Pelizza (2016b) portrays data friction as conflicts revolving around finding the best configurations of actors, institutions, and resources to ensure dependable data. As she emphasizes, even in complex systems designed to mitigate friction, complete removal is often unattainable; instead, the associated costs tend to shift to alternative actors, organizations, or material forms. Consequently, we may hypothesize that data frictions concerning re-identification present associated costs, such as organizational labor, interpretive activities, task complexification.
Identity data takes on diverse forms as it navigates through different actors and organizations, and these transformations may entail associated costs when utilized for re-identification purposes. I propose that the concept of data friction can be extended to the movement of identity data across organizations and different material forms. For instance, as identity data transitions from a physical passport to a digital database record or moves between the systems of different organizations, barriers may emerge, leading to friction in the smooth movement of identity data and, consequently, re-identification. Regulatory constraints undoubtedly influence the movement of identity data between organizations. However, discrepancies may also arise due to variations in naming conventions, differences in date of birth formats, or inconsistencies in the use of characters like hyphens or spaces in surnames across organizations. To illustrate, let us consider my experience while applying for a Russian visa in 2018: my passport information was copied into various systems, leading to an error where my second name was mistaken for a patronymic in the application. Moreover, my first name was inadvertently listed with the letter “v” instead of “w” in the machine-readable zone of the visa due to the absence of the letter “w” in Cyrillic. These confusions stemmed from differences in naming conventions and ambiguities in the transliteration process. Even seemingly minor discrepancies like this can create complexities in the re-identification process.
Mechanisms aimed at mitigating data friction in the context of re-identification are likely to bring about shifts in associated costs. For instance, one hypothesis could suggest that the circulation of identity data and the presence of data friction is closely linked to the proliferation of duplicate records. Consider the scenario where various organizations share a common database: disparities in identifying individuals, like variations in naming conventions or data formats, could lead to registering multiple entries for the same individual. In this and similar cases, streamlining data frictions by integrating data matching tools into bureaucratic systems simultaneously redistributes costs. Integrating such tools, for example, might necessitate organizational adjustments, as staff may need to allocate additional resources to manage other aspects of re-identification, such as the labor-intensive task of detecting and resolving identity discrepancies.
This section has reviewed literature that has been instrumental in conceptualizing re-identification as an iterative bureaucratic practice, emphasizing its materiality and performativity dimensions. The conceptualization of re-identification as a bureaucratic practice underscores its significance within the interactions of bureaucratic organizations with applicants, particularly as these interactions become increasingly digitized and automated. As a bureaucratic practice, it underscores the potential links of re-identification with the exercise of discretion by bureaucrats in routine re-identification practices at the street-level and screen-level. Re-identification can be further contextualized at the system-level within the realm of the materiality and performativity of devices, ultimately influencing the evaluation of data, individuals, and organizations, shaping their credibility and reliability.
Additionally, delving into the technical mechanisms of data matching not only uncovers operational intricacies but can also serve as a means to gain insights into the diversity of re-identification processes. Integrating data matching tools within bureaucratic systems with the aim of reducing data friction can inadvertently shift the associated costs. As such, the literature discussed here supports the idea that the re-identification of individuals throughout bureaucratic processes and data infrastructures is a crucial but understudied area of research. An iterative approach to identification underscores that re-identification is not a one-off event but an ongoing, multifaceted process spanning diverse bureaucratic tiers. It encompasses street-level interactions, screen-level engagements, and system-level operations, unfolding across both spatial and temporal dimensions. This approach enables us to hypothesize that data friction is an inherent element within these iterative identification processes, potentially leading to less-than-optimal re-identification outcomes that entail organizational costs. The next section will describe the empirical case and the methods used to investigate re-identification.
5.3 Case and method: Empirical analysis of the interplay between data matching systems and applicant re-identification
The investigation into re-identification within migration management draws upon data collected through fieldwork conducted in person and remotely between July 2020 and July 2021. While a comprehensive methodological framework is outlined in Chapter 3, this section offers additional specific details tailored to the context of this chapter. Throughout this fieldwork, I established a collaborative partnership with the Dutch company WCC Group, specializing in developing data matching and deduplication software. In the context of this chapter, the focus will be on the software’s use by the Netherlands’ Immigration and Naturalization Service (IND). The IND, entrusted with responsibilities such as processing residency and nationality applications, utilizes the ELISE software for searching and matching applicants’ identity data within the back-office system and also assists in managing data anomalies, such as duplicate records.
The fieldwork delved into applicant re-identification procedures at the IND, specifically examining their interplay with WCC’s “ELISE ID platform.” By design, the matching system aims to circumvent errors in both the database and the search criteria. For instance, it can automatically accommodate instances where the date of birth is incomplete or date and month values have been inadvertently interchanged in the search query or database records. Using the ELISE system thus facilitates re-identification when discrepancies may arise from difficulties in matching personal data from different locales, scripts, and cultural contexts. The re-identification of an applicant through a search, based on factors like their name, nationality, and date of birth, results not in a simple roster of exact matches but rather a compilation of applicants with an associated value signifying the likelihood of a match between identity data records. In short, the data matching engine integrates diverse algorithms into a cohesive system that aims to address uncertainties in identity matching, thus supporting the IND’s operational processes.
Methodologically, the research considered discrepancies between tool design and practical usage. This was accomplished by comparing how different organizational actors within the IND employ data matching capabilities in their daily re-identification tasks. The study hypothesized that for re-identification to be effective, for IND staff to use the search and interpret the results effectively, there must be some alignment between the system and its users. The research placed a specific emphasis on understanding the challenges faced by IND staff while employing search and match functions in IND’s systems, revealing underlying assumptions and expectations of the data matching system. Indeed, discrepancies between intended use and actual application might underlie challenges in re-identification.
5.3.1 Data collection
The data collection process was facilitated by my involvement as a temporary member of WCC’s ID team. This collaboration enabled me to access essential technical documentation and engage in informative meetings, including some conducted on-site at the company’s headquarters in Utrecht, The Netherlands. The collected technical documents regarding integrating the ELISE data matching system into the IND systems can be classified into three categories. Firstly, some documents cover the ELISE system’s overarching technical specifications. Independent of any specific organizational implementation, these documents provided insights into the data matching software’s overall design and intended applications. Secondly, a trove of technical documents, meeting minutes, and presentations delved into the precise implementation of the ELISE system within the context of the IND. These resources helped analyze the search and match software integration into the IND’s systems. Thirdly, the collected documents encompassed public communications, such as online news aimed at ICT professionals and official reports like government audit findings. These sources contributed an additional layer of context regarding the evolution and establishment of the IND’s information system. The initial reading of these documents, accompanied by annotation and note-taking, served as a jumping-off point for structuring the questions to be asked during the interviews with IND staff.
Following the document analysis, I conducted semi-structured interviews to gain insight into the development and use of the search and match tools. As detailed in Chapter 3, interviewees can be divided into two main groups based on their themes and used different protocols for each group. The first group was centered on IND staff — the users, whose duties include looking up and matching identities in their databases, which necessitates using the ELISE ID platform. The analysis in this chapter mainly draws on data from interviews with IND staff and notes from the briefing meetings with WCC ID team members. The second group was WCC staff involved in the software’s development, deployment, and maintenance as designers. Although these interviews with WCC staff provided additional context about ELISE, they are not directly featured here and will be more important for Chapter 6. With the aid of the ID Team, initial contact with the IND was established, yet pandemic constraints necessitated interviews via online meetings or phone calls. In five interviews, each spanning roughly an hour, participants shared their experiences with the search and match tools at the IND.
The interview protocol for IND staff was designed to explore various aspects of re-identification within the IND organization. Interviews began with general inquiries about the interviewees’ roles within the IND, providing context for understanding their experiences and tailoring subsequent questions. The initial questions centered around three key factors influencing the searching and matching of applicant data. These factors are how search queries are formulated, the computation of matches, and the handling of search results. Regarding the first factor, questions included IND personnel’s approach to formulating search queries, including the data categories they input, their knowledge of data elements that yield better search results, and their utilization of match features such as wild cards. For the second factor, questions were tailored to uncover their expectations regarding the match results and their understanding of the match engine’s functionality. For the third factor, the questions probed on their processing of search results, addressing their perception of result quality, the ranking of matches, and the interpretation of match scores. Next to those questions, the protocol inquired about duplicates and the deduplication process, inquiring about the criteria used to identify duplicates and the organization’s approach to resolving them. Lastly, the protocol investigated the participants’ use of additional systems and data to support the re-identification process. Overall, the interview protocol aimed to provide comprehensive insight into the search and match procedures and the challenges IND personnel face in re-identifying applicants.
5.3.2 Data coding and analysis
For analyzing the fieldwork data, I followed standard methods for coding and analyzing qualitative data. After collecting and preparing data from documents and interviews (including transcription), I coded and analyzed the data using the computer-assisted qualitative data analysis software ATLAS.ti. The data coding and analysis drew inspiration from the three interconnected steps of the “Noticing-Collecting-Thinking” (NCT) method by Friese (2014), which follows a standard qualitative data analysis but is tailored for the ATLAS.ti software. The Noticing phase involved both deductive codes and openness to inductive insights from the data. These codes were reviewed and organized into similar categories in the Collecting step. The third step, Thinking, led to identifying patterns, processes, and typologies among the developed codes. Figures 5.2 and 5.3 provide a simplified overview of this process, illustrating broad deductive themes on the right, more inductive findings in the middle, and representative interview excerpts on the left.
In more detail, the first step of the data coding process began with deductive coding, aligning with the key factors influencing the search and matching of applicant data, as outlined in the interview protocol. These factors encompassed the formulation of search queries, match computation, and handling of search results. The data coding utilized several of these predefined codes, falling under broad categories like “search query,” “search engine,” and “search results.” This coding method began by applying these predetermined codes to relevant excerpts. However, these codes were subsequently refined through an inductive approach, recognizing patterns within the interview excerpts.
The second step of refining and collecting codes proceeds through adding a colon “:” to the code and names to introduce inductive sub-codes. For instance, “search query: use of data: amount of data available” corresponds to a deductive category concerning the types of data employed in crafting search queries (“search query: use of data”). The inductive aspect (“available data”) emerged from the interviews and was consistently used for quotes referencing the quantity of data available concerning formulating search queries for re-identifying applicants.
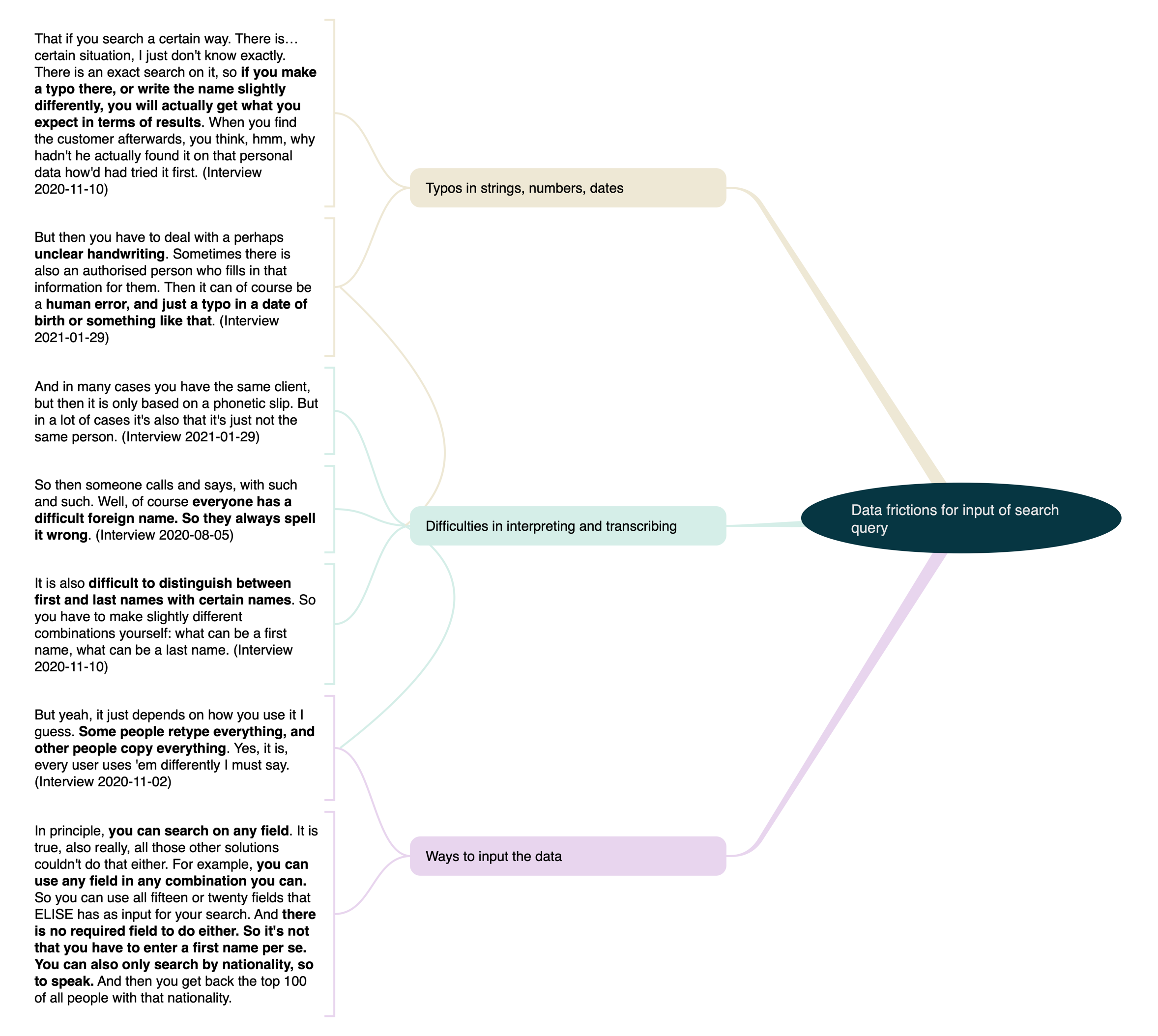
Figure 5.2: This diagram shows how friction with search query input were found by analyzing interview data.
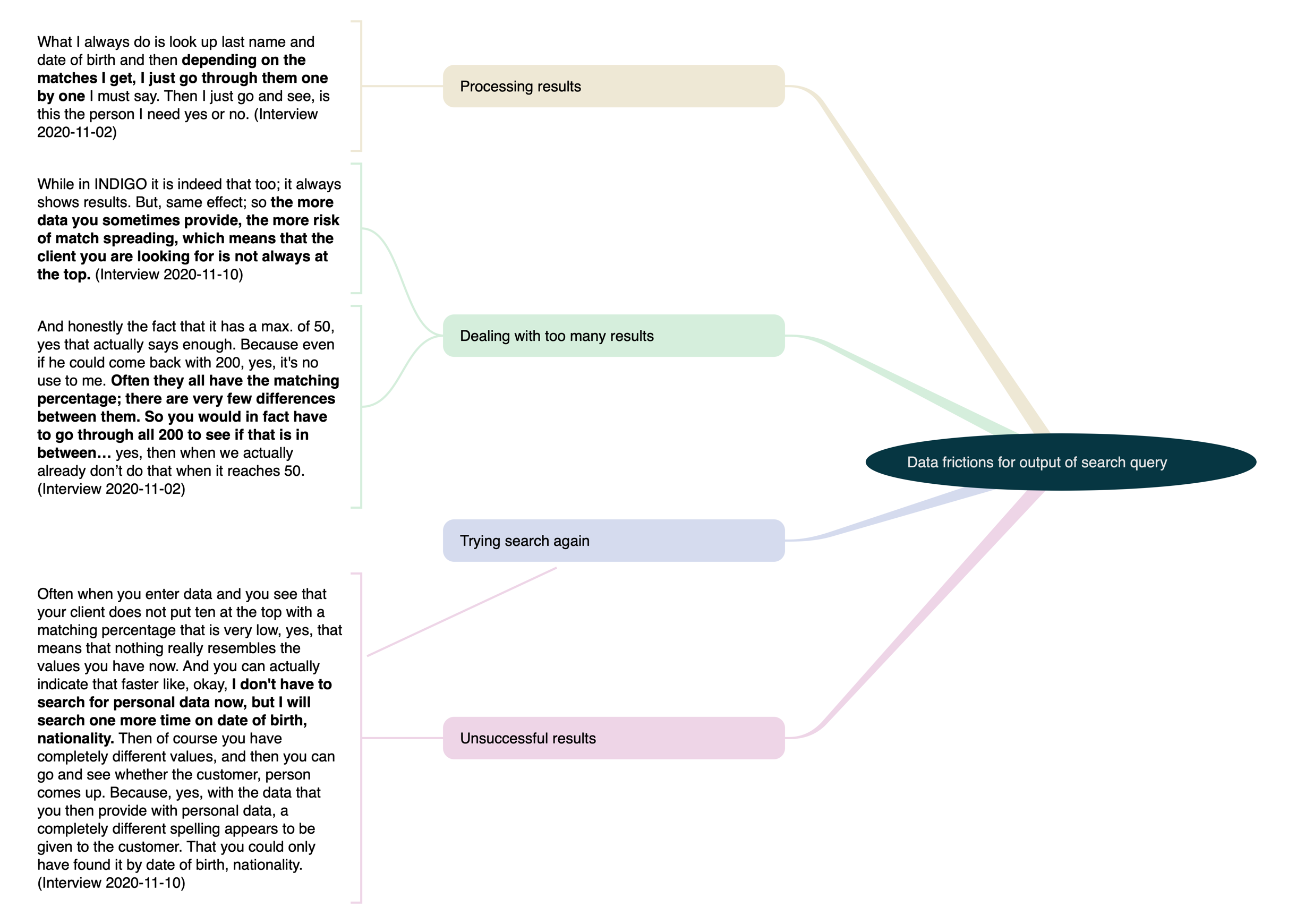
Figure 5.3: This diagram shows how friction with interpreting search results were found by analyzing interview data.
The third step of the process involved further discerning patterns, processes, and typologies within the developed codes. Two illustrative examples encompassed an in-depth analysis of friction associated with search query input and output, visualized in Figures 5.2 and 5.3. In the diagram illustrating search input, three main challenges were identified, each linked to underlying codes associated with challenges in constructing search inputs due to typographical errors in strings, numbers, and dates; complications in transcribing or interpreting data; and uncertainties about how data should be input (e.g., determining the extent of data to input and which combinations to use). Conversely, the figure illustrating search output captured different broad types of challenges, again connected to more specific underlying codes tied to processing results, including instances where the output yielded an excessive or insufficient number of results, as well as instances where the results were unexpected.
5.4 Exploring the designed infrastructure for identifying applicants
This section starts the empirical analysis by situating the re-identification of applicants33 at the Immigration and Naturalization Service (IND) within its software architecture and inter-organizational frameworks of the Netherlands’ migration policy. Understanding the organizational and software architecture helps situate the intricacies and challenges of the IND’s re-identification processes. The IND’s operations rely on its information systems called INDiGO, designed to manage the identification and registration of applicants applying for various purposes, including residency or naturalization. Additionally, INDiGO interfaces with various partners and stakeholders within the migration chain whose systems and databases also play a role in the IND’s processes. This initial examination of the organizational and software architecture will provide the foundation for the following sections, in which this architecture and software designs will be compared to their practical implementation.
5.4.1 Migration chain and identifying chain partners
The processes and practices involved in re-identifying applicants for the IND need to be understood within the larger context of the information infrastructure for handling foreigners in The Netherlands. The IND is just one link in the “migration chain” (migratieketen), a collaboration between various governmental and non-governmental organizations in The Netherlands. Each link in this chain, known as a “chain partner” (ketenpartner), is responsible for different processes foreign nationals in The Netherlands go through, including entering the country, obtaining a residence permit, naturalization, and departure or expulsion. These partners exhibit interdependence since their decisions often necessitate information from others, facilitated by an interconnected information infrastructure.
The information infrastructure of the migration chain can be traced back to a subfield of information science called “chain computerization” or keteninformatisering in Dutch, which has been influential in Dutch academia and government digitalization in The Netherlands (e.g., Grijpink 1997). Chain computerization pertains to the information infrastructure of the networked chain of interdependent organizations without a formal hierarchy (Oosterbaan 2012). These entities collaborate and exchange information to execute a shared process, exemplified in this context by the handling of foreign individuals within the Netherlands. The migration chain, as outlined in Zijderveld, Ridderhof, and Brattinga (2013) that describes its architectural framework, defines principles and objectives aimed at enhancing information exchange. It addresses identification challenges, particularly those contributing to duplicate registrations among chain partners, and constitutes an important focal part within the architecture.34
Foreigners within the migration chain are assigned a unique identifier known as the “v-number,” which is used for identifying individuals throughout the chain. This unique identifier is issued through the Basisvoorziening Vreemdelingen (BVV) system, functioning as a centralized repository for sharing and consulting information about foreign nationals among the various chain partners. Upon the first contact with a foreign national, the relevant organization must ensure that the individual has not been previously registered and, consequently, already possesses a v-number. The BVV database can be updated and enriched with identity data, travel information, identity documents, biometric characteristics, and status data, such as asylum application outcomes, originating from the chain partner’s systems. Even though every partner in the chain has their database and information regarding migrants, the use of BVV and the v-number can enable the linkage of information by utilizing the v-number as a shared and unique identifier (ICTU 2015).
The processes of first registration and identification of foreign nationals are further directed by the “Protocol identification and labeling” (PIL, “Protocol identificatie en labeling” in Dutch) The various chain partners use this protocol; it standardizes the process of identifying and registering foreign nationals as a way to ensure that “unique, unambiguous personal data of optimal quality are available in the migration chain” (Ministerie van Justitie en Veiligheid 2022, 9). As implied by its name, the protocol also includes a labeling provision. If someone is hesitant or unwilling to reveal their name, they will be assigned a label. This label will serve as their name and contain details about their gender and the date, time, and place of registration. For example, the label could be NN regioncode sex yymmdd hhmm. Therefore, the protocol can be interpreted as being designed to minimize identity multiplicity and streamline subsequent re-identification by providing clear guidelines for recording individuals’ data. Nonetheless, as elucidated later in this chapter, the presence of individuals possessing multiple v-numbers indicates that this ideal scenario may not always hold in practice.
The key takeaway from the architecture of the migration chain is that the unique identification of individuals is deemed crucial for re-identifying foreigners in The Netherlands among all the chain partners. Specifically, the IND, as one of the chain partners, relies on the BVV systems and v-number for effective identification and re-identification of applicants. Through this re-identification process, the IND is tasked with confirming whether an applicant has not already been initially registered by other chain partners such as the “Vreemdelingenpolitie” (national police) or the “Koninklijke Marechaussee” (national gendarmerie). Next, we will delve deeper into the systems employed by the IND and explore further their interactions with the BVV and other chain partners.
5.4.2 Unpacking the IND and INDiGO infrastructure
Shifting the focus from the broader discussion of the identification of foreign nationals in the migration chain, this section delves into a more specific examination of how applicants are identified in the information systems employed by the IND. The central pillar of this information infrastructure for application and identity management is the INDiGO system.35 The implementation of the INDiGO was part of a more extensive digitization project and data transfer from a previous system called INDIS. The distinct manner in which the upgraded system technically compartmentalizes various facets of organizational operations is of particular significance. This division is primarily manifested in the separation of policy implementation, which involves the application of business rules aligned with the Dutch Aliens Act, from information management tasks, including data storage, searching, and matching (KPMG IT Advisory 2011).36
The information infrastructure of the IND, as outlined in Figure 5.4, can be characterized as a form of system-level bureaucracy (Bovens and Zouridis 2002), given that INDIGO places significant emphasis on information management and the automation of decision-making in the processing of digital dossiers.37 At the system level, the identification of applicants unfolds through automated data exchanges, where applicants are re-identified in processes to update their applications and dossiers. Re-identification also extends across multiple bureaucratic tiers, spanning the street- and screen-levels. At these levels, IND staff, both at the front and back offices, interact with the graphical interfaces of the information systems to confirm and verify applicant identities while processing their applications. The following section will examine how INDiGO utilizes the ELISE software for searching and matching applicant data across all these bureaucratic levels.
Figure 5.4: A schematic representation of the IND’s information infrastructure, illustrating its role in facilitating tasks related to application interactions, and inter-organizational collaboration with MC partners.
5.4.3 Applicant re-identification and matching with ELISE software in the INDiGO system
Throughout the evolution of INDiGO, the ELISE software for searching and matching applicants has been applied in various scenarios, which can be categorized into the following three cases. First, the ELISE software was initially used during the transition from the old IND information system (INDIS) to the new INDiGO. During a transition period when INDIS and INDiGO ran in parallel, the IND used the ELISE software to migrate legacy data by re-identifying matching applicant identities between the two systems.38 The ELISE software’s second and most prominent use is to facilitate applicant data searches. While the underlying case management system provides a “traditional” search, this was deemed insufficient because it would fail to return results when search criteria are too strict or contain errors. For this reason, the ELISE system was added to use the software’s fuzzy search algorithms to provide more advanced and reliable searching capabilities (Interview 2020-08-05). Third, the ELISE software searches the database for possible duplicate applicant data. The software attempts to match all recently created applicants to all other applicants in the database. Potential duplicate matches that meet specific criteria will then be flagged and investigated further. In all three uses, the software calculates match scores calculated by the software based on the likelihood that an applicant in the database meets the given search criteria.39
Following my analysis of the technical architecture and utilization of ELISE in INDiGO, as detailed in design and technical documents, I suggest categorizing the searching and matching into three essential components: query input, processing by the matching engine, and results output. Designed as a generic and decontextualized component within the INDiGO system architecture, ELISE is intended to function independently, receiving input from various sources. This input might originate from the INDiGO graphical user interface or other automated processes. According to the documentation, the system is designed with the recognition that both search queries and database records can contain errors. This design thus accommodates scenarios such as typos, the inadvertent swapping of first and last name fields, and similar errors, whether they are already present in a record within the database or introduced during the formulation of a search query. Within INDiGO, the search and match functionality operates without specific user distinctions, instead relying on the ELISE system as a data matching service configured universally and integrated into various components of the INDiGO system.40 This prompts the question: what implications do this absence of user-specificity have for the re-identification practices of the IND?
Per the system’s technical documentation, queries originating from IND end-user applications are channeled to the ELISE service, which employs diverse algorithms to compute matches. In practice, the data matching engine assesses the similarity between the input query and all database records, generating a corresponding similarity score. The computation of this match score can be adjusted through system configurations, allowing certain factors to be weighted more or less significantly. The matching process encompasses deterministic data matching algorithms that calculate similarity by considering variations in name spellings, utilizing methods such as name initials and even intelligently accounting for transposed numbers, evident in dates of birth or identification numbers. Additionally, the engine leverages name data databases to facilitate advanced matching techniques based on rule-based or domain knowledge, such as accommodating name transliterations and recognizing variations like “Aleksandra” and its diminutive form “Ola.” Furthermore, the system incorporates probabilistic matching mechanisms, including a feature termed affinity matrices, which involve attributes like the “soft matching” of birth years within a reasonable range. For example, if a search specifies a birth year as 1990, the system can be configured to consider birth years slightly earlier or later, covering a span like 1988 to 1992. By employing these diverse matching features, the system assigns a match score that gauges the likelihood of a match between the search query and the corresponding database entry. These system functionalities raise questions about the influence of ELISE on the re-identification expertise of IND personnel, potentially shifting the locus of expertise from street-level bureaucrats to the system.
The system returns a set of records ranked based on their closeness of match to the query, as opposed to offering a single match in response to a database lookup. By design, the data matching process always yields results, even if no exact match is found. The number of matches returned is also adjustable within the system. It is important to note that, due to its modular structure, the results are sent back to the point of origin of the search, such as being displayed through the INDiGO graphical user interfaces. Consequently, the searching and matching service has limited insight into where within the INDiGO system and process the call is initiated or who is making the query. Subsequent sections will explore how this user-agnostic approach aligns with the actual usage patterns for the IND’s re-identification practices.
5.4.4 Architectural and system design influences on applicant re-identification at the IND
This section has described architectural and system design elements of the IND composite information environment, and highlighted how they can influence the re-identification of applicants within the IND. The discussion has delineated two main dimensions that shape the IND’s data infrastructure and subsequently impact the re-identification process.
Firstly, operating as a node within the migration chain, the re-identification practices of the IND are not isolated but rather interconnected with the diverse partners comprising the migration chain. During the initial interaction with an applicant, the agency is tasked with validating whether the individual is already within the records of the migration chain. The v-number, functioning as a unique identifier for foreign nationals in the Netherlands, facilitates the process of re-identification and linkage of applicant data across the chain partners. However, re-identifying applicants will become considerably more intricate when this identifier is unavailable. The introduction of mechanisms like the PIL strives to establish standardized initial registrations to facilitate smoother re-identification in subsequent stages. In the following section, we will examine the practical aspects of these interactions and delve into the complexities of re-identification, including the interaction between different systems and the challenges faced by IND personnel.
Secondly, the IND’s strategies for re-identification are intertwined with the ELISE data matching system. This system acts as an intermediary in the re-identification of applicants, addressing various uncertainties surrounding identity data. Positioned as a loosely coupled module within the broader INDiGO system and its accompanying databases, ELISE aims to facilitate the process of applicant re-identification. The system’s design acknowledges the inherent uncertainties in search queries and database accuracy. In the upcoming section, we will delve into the empirical data gathered from fieldwork, elucidating three distinct types of data friction that can hinder re-identification, stemming from disparities between the intended designs of systems and their actual practical utilization.
5.5 Putting the design into practice: Investigating the practical application and challenges in the IND’s identification processes
5.5.2 Friction 2: Balancing precision and accuracy in the movement of identity data
This section, along with the next one, will center on three primary aspects of the search and matching process hypothesized to play a role in the successful re-identification of applicants: the formulation of search queries, the calculation of matches, and the handling of search results. This division aligns with the interview protocol’s structure, as elaborated in the section on data collection. The analysis will commence by exploring the challenges associated with formulating search queries. As emphasized in the design discussion, the absence of user-specificity in the search and match functionalities design means that these functions are intended to operate uniformly and generically for all users within the IND, without distinct configurations or adaptations tailored to specific user roles or preferences. This design choice might have implications for re-identifying applicants at the IND, as it raises questions about how effectively the system can cater to the diverse needs and practices of different users and departments involved in the re-identification process. The interview excerpts and scenarios presented in this and the subsequent sections are selected as they are deemed the most illustrative of potential data friction that could impede smooth re-identification when using the search and matching tools, as revealed through the analysis.
To start, it is essential to acknowledge that for respondents the lack of user-specificity is not a prominent concern, primarily due to the simplicity of formulating search queries. Participants underscored that the most fundamental and frequently utilized method for searching and re-identifying applicant data within the IND adopts distinct identifiers like the v-number. For instance, applicant re-identification might occur while processing new information related to an ongoing application in back-office settings or direct interactions with applicants at front-office counters. In these cases, the procedure is ongoing at the IND, and staff can thus execute such searches on the system relatively seamlessly, as the applicant is already known to the IND and the v-number can be employed.
However, as highlighted in the subsequent interview excerpts, IND staff members frequently encounter situations where formulating seemingly straightforward search queries becomes complex due to various factors. These scenarios often involve dealing with intricacies stemming from data inaccuracies, misunderstandings, and human errors, especially when data is received or input through diverse means like handwritten documents or phone conversations. In specific departments, such as those handling handwritten documents sent via postal services, employees often grapple with accurately deciphering and transcribing handwritten data, consequently introducing an element of uncertainty into the re-identification process. To illustrate, the following example from an interviewee sheds light on the challenges arising from processing handwritten application forms and managing errors or ambiguities in the provided date of birth:
But then you have to deal with perhaps unclear handwriting. Sometimes there is also an authorized person who fills in that information for them. Then it can, of course, be a human error and just a typo in a date of birth or something like that. (Interview with IND staff member, January 29, 2021)
Another instance of this issue involves the potential confusion between first and last names. IND personnel may encounter situations where the data provided by applicants does not clearly distinguish between these two components. This ambiguity can create uncertainty for the IND staff when using the search fields in INDiGO, as highlighted by the following interview excerpt:
It is also difficult to distinguish between first and last names with certain names. So you have to make slightly different combinations yourself: what can be a first name? What can be a last name? (Interview with IND staff member, November 10, 2020)
There are instances where the perceived match between applicants might originate from phonetic errors or other subtle variations. For instance, envision a situation where a name like “Rousseau” is misheard or misspelt as “Russo,” resulting in what the interviewee below refers to as a “phonetic slip”:
In many cases, you have the same applicant, but it is only based on a phonetic slip. But in a lot of cases, it’s also that it’s just not the same person. (Interview with IND staff member, January 29, 2021)
As previously outlined, the ELISE data matching system is intentionally designed to address such potential errors in search query inputs, aiming to account for possible typos, mixed names, and similar variations during matching. Nonetheless, when I inquired about this aspect during the interviews, there appeared to be a general lack of specific knowledge about how this mechanism functions for search query inputs. However, as noted in the following excerpt, the mechanism that accounts for search input could occasionally lead to confusion in the search results – a subject we will revisit in our subsequent discussion regarding the computation of matches and the handling of search results.
[I]f you search a certain way. […] I just don’t know exactly. There is an exact search on it, so if you make a typo there or write the name slightly differently, you will actually get what you expect in terms of results. [But] When you find the applicant afterwards, you think, hmm, why hadn’t it actually found it on that personal data I had tried first? (Interview with IND staff member, November 10, 2020)
The formulation of search queries can become more ambiguous and error-prone, as evidenced by the excerpts from IND staff members. These issues are particularly notable when dealing with phone calls or handwritten documents, where elements like v-numbers, dates of birth, or applicants’ names might contain typos or be challenging to read. In these contexts, data friction arises as identity data transitions between different material forms, arising from difficulties in comprehending or accurately transcribing information. Consequently, this can hinder the successful re-identification of an applicant. Interestingly, this challenge aligns with the design of the data matching system, which anticipates potential errors in search queries and compensates for such uncertainties. Here we can see how the data matching system thus functions as a mechanism to alleviate data friction as identity data shifts across various media. However, there is a potential disconnection between users’ expectations of search input accuracy and the system’s ability to accommodate errors and uncertainties in the query.
The findings on the formulation of search queries contribute to our understanding of re-identification in two ways. Firstly, they underscore the distinction between different forms of data employed in the re-identification process. While certain data, such as identification numbers like v-numbers, demonstrate a higher capacity to traverse various material forms and actors, personal data like names and birthdates are more susceptible to discrepancies, errors, and interpretive challenges. Nevertheless, both data types are vulnerable to errors, whether through typos, ambiguities, or variations, potentially complicating the re-identification process. Secondly, the findings indicate a disparity between the system’s intended flexibility, designed to accommodate errors and uncertainties in search queries, and users’ assumptions regarding the accuracy of their inputs. Users may expect accurate input, while the system automatically rectifies any errors they make in their queries. This disparity can create friction in re-identification, and introduce frustration and inefficiency into the re-identification process when the system includes unanticipated results. This finding underscores that while the mechanism designed to mitigate data uncertainty in formulating search query inputs is effective, it can inadvertently lead to ambiguities, particularly when users are unaware of its functions.
Another aspect of search formulation that warrants investigation is the amount and combinations of input data required for successful re-identification. While the ELISE data matching system is designed to operate optimally when the search query includes as much information as possible, the interviews also probed into the specific combinations and amounts of data actually utilized in search queries. However, this aspect is intricately interlinked with the expectations surrounding search computation and the subsequent results, which will be discussed in the subsequent section.
5.5.3 Friction 3: Deciphering opaque match results for successful re-identification
Continuing the investigation of the three aspects thought integral to the re-identification of applicants through the search and matching process, this section now delves into the practical complexities associated with match calculation and the subsequent handling of search results. By delving into these aspects, we can address the questions and hypotheses that emerged during the discussion of the system’s design. By looking at these two aspects, we can examine the implications of ELISE’s functioning on applicant re-identification, considering its potential to complement or replace the re-identification expertise of IND personnel. Additionally, we can analyze how the presentation and handling of search results can either facilitate or hinder the re-identification of applicants.
In general, interviewees showed an awareness of these data matching features during their searches. However, there was also a prevailing sentiment that additional features might operate beyond their explicit knowledge. When probed about more advanced search functionalities, one interviewee indicated that while users may not fully comprehend the intricacies of the search process, they can typically accomplish applicant re-identification. The following quote captures a common sentiment among interviewees — a sense of uncertainty regarding the exact calculation of the match score:
[N]ow and then it is very hazy how [the search] exactly works. For example, sometimes a letter seems to be more important than at other times, depending on where it is located. But you usually see if you misspell such a letter that you then do not get a hundred per cent hit. But then you still get sixty per cent or so. In some cases, it is also higher than that percentage. But you usually find [the applicant]. (Interview with IND staff member, August 5, 2020)
As mentioned previously, the ELISE data matching system is designed to work best when the search query includes as much information as possible. By doing so, the data matching algorithms can utilize all this input data to calculate match scores. However, as the comments below demonstrate, there was a mixed sentiment among interviewees regarding the usefulness of providing more input data. While some perceived that additional data did enhance results, for others, it did not necessarily guarantee improved outcomes:
If you have more data, you look at what more you can put in it. So you’re actually trying to make it as broad as possible. If you have a date of birth, you have a street name, or you have something else, to increase the matching percentage. And then you actually also look — if there are multiple search results — then you actually look first at the highest matching percentage.
My experience with searching for personal data is that the more data you enter, the more difficult the result will be. And the worse the result actually gets. So I often build it up. I [input] less data, and if necessary, I add some data if there are too many results. (Interview with IND staff member, November 10, 2020)
One interviewee aptly described this situation, stating that INDiGO staff sometimes feel compelled to “play” around with the search tools until they successfully identify the desired applicant.
[…] what you often see in how they work is that hey, they use it first with one type data. And if they still get too many results, or they don’t see it, they try with an extra piece of data. Or they try it with another kind of data. So you see, to find a person, they sometimes do five searches in a row. Also, a little, OK they could enter everything at once, but you can see they play with that a little bit. (Interview with IND staff member, August 5, 2020)
The interviewee’s statement hints at the possibility that IND personnel engage in various permutations of data categories when conducting searches. On the one hand, this highlights a tension between the expected use of ELISE, which encourages providing as much search input as possible, and the practical experience that entering excessive query information might occasionally worsen results and introduce ambiguity in re-identifying the correct applicant. However, another interviewee sheds light on an alternative approach to mitigating uncertainty in re-identification. This method involves experimenting with different combinations of data or deliberately omitting certain information. Remarkably, by not inputting certain details, these omitted elements can later be utilized to cross-check results, potentially reducing uncertainty in re-identification. The interviewee outlined their process as follows:
And there is also a kind of self-check in [the search process]. So I often search by first name, last name; to start with. […] But I often try not to do too much and see if that result is there. And on that basis, OK, the date of birth also matches the date of birth that I have. So I don’t always deliver what I have available as information. But I also partly use it as a checkpoint for the search result that I then get to the top. It also works a bit more efficient for me. Because it makes no sense to enter much more data. Because you can find the applicant anyway, also sufficient on the basis of first and last name. And you get insight so that you immediately know that you have the right one. (Interview with IND staff member, November 10, 2020)
Upon sharing this scenario with a senior developer from WCC, their surprise at the idea of deliberately withholding input data to reduce uncertainty underscored the friction that can emerge between the intended design of a system and its actual real-world application. For IND personnel, the data matching process can sometimes feel like a black box, amplifying the uncertainty around determining the optimal input of information into the data matching system. Choosing the appropriate amount of information to provide, what to include or exclude, and the potential consequences of different combinations requires additional efforts in the re-identification process, pushing staff to allocate more time and resources to refine their searches.
The complexity surrounding the functioning of the matching process came to the forefront when matches were generated utilizing information stored in “historical fields.” These specialized fields exist within the IND’s data model to accommodate the storage of multiple values for the same data category, thereby allowing for the retention of various historical information about an individual. This aspect was highlighted during interviews with multiple participants who grappled with comprehending the logic behind including certain applicants in the match results. The confusion arises from the fact that only the most recent values from these fields are considered in the search results. To illustrate, imagine a scenario where a search is conducted using an individual’s pre-marital name, yet the results display an applicant with an entirely different post-marital name due to a subsequent name change. An interviewee aptly captured the complexity of deciphering matches based on historical data in the following manner:
And what is actually very interesting in [the case of the IND] is that someone does not just have one address but can have several addresses, for example, or even several names. And he may have changed his name, for example. So then the old name is also saved. You actually have a primary field, for example, for name or address. And you have historical fields. And they are all searched with ELISE. […] So we actually have the history of every field. That can contain one value, but it can also contain ten values. And if you match that. I think that there is also an interesting point with user expectations. […] I think they’re not always aware of that. That if they find someone, it can also be based on an old date of birth, which has been entered incorrectly, or based on an old name. (Interview with IND staff member, August 5, 2020)
When specific categories within the IND’s data model contain multiple values, it can both facilitate and hinder re-identification. On the one hand, this approach makes it easier for the agency to re-identify individuals by considering both their current and past data. However, this flexibility also poses a challenge. Scrutinizing matches using historical data becomes more complicated, adding to the workload of personnel. During an interview, one interviewee shared that they acknowledged that such matches are likely to happen and emphasized the importance of carefully examining the results to understand why the match was included:
[…] experience has taught me that often the name has been changed. So there is also a history; so if someone has a different name, if a name is changed — and that is sometimes changed considerably — then you will indeed get it as matching. And when clicking through on the history of the name, you see that the logic comes from there, that it knows from history that it was called differently. And that is why it is shown. That is my experience. (Interview with IND staff member, November 10, 2020)
Likewise, a comparable challenge arises when matching relies on interconnections between applicants or other entities. The INDiGO system also records data about various entities, such as lawyers representing applicants or affiliated organizations. Hence, as elucidated by the subsequent interviewee, an effective strategy for re-identifying an applicant entails exploring linkages within the system. Their strategy also involves searching for applicants registered under a specific affiliated lawyer if a direct search for the applicant proved unsuccessful:
Then you try to see if you cannot find another way to find the applicant. It may sound a bit strange, but sometimes you can see […] to which lawyer it was submitted, via which lawyer it was ever submitted. And then you can look through the lawyer and which applicants he has under him. That way, you can also indirectly find out which applicant it is. But that is the difficulty when it comes to finding applicants. (Interview with IND staff member, November 10, 2020)
By design, the top results with the highest match percentage should ideally present the most relevant matches, making the matching applicant readily identifiable among these top results. However, in practice, this ideal scenario is not always realized. Responses from the interviewees reveal that users have developed various strategies to decipher results, particularly in distinctive cases. As one interviewee succinctly put it, IND staff are, at times, “actually trying to fine-tune [the search] so [they] can get the right person up” (Interview 2020-11-02). This phrase highlights challenges in re-identifying applicants and ensuring their appearance among the foremost search outcomes. In this context, it is reasonable to consider that re-identification issues emerge when a disparity arises between the user’s intended goal (successfully re-identifying an applicant) and the actual outcome presented by the system (a collection of search results). These difficulties introduce a third form of data friction in the re-identification process, stemming from the interplay between the system’s ranking of search results and the efforts of IND staff to re-identify and retrieve applicants’ data. This friction is effectively demonstrated through the struggle to retrieve an applicant’s data and ensure its prominent positioning within the search results.
These findings on the practical challenges of calculating matches and the subsequent handling of search results further add to our understanding of re-identification. The somewhat enigmatic nature of result calculation highlights a nuanced interplay between human interactions and the capabilities of data matching tools that both facilitate and hinder re-identification. While the interviewees exhibit a certain degree of understanding regarding aspects of the data matching system, such as basic fuzzy search techniques, it becomes apparent that the system employs additional features and autonomous functionalities that often operate beyond their explicit awareness. On the one hand, these features can streamline re-identification efforts, even if the users are not fully aware of the underlying mechanisms. Conversely, disparities in understanding can introduce an additional layer of intricacy, prompting staff to invest substantial time and resources in refining, comprehending, and critically assessing search results to ensure accuracy and dependability. As we will see next, the repercussions of failed re-identification extend beyond these challenges, often triggering issues such as creating duplicate records, which will be explored in the next section.
5.6 The costs of failed re-identification: Duplicates and the labor of deduplication
As established in the preceding sections, the interplay between the design of standardized identification practices and the utilization of data matching technologies can introduce forms of data friction in the re-identification process. This section will explore their broader consequences, focusing on a twofold outcome of unsuccessful re-identification: the presence of duplicate records for applicants and the ensuing labor-intensive deduplication process.
5.6.1 The deduplication memo and resolving identity duplicates
As highlighted previously, the issue of duplicates arises when an organization has multiple disjointed database entries for the same individual, a challenge that many organizations commonly face (Christen 2012; Keulen 2012). As we have explored forms of data friction influencing re-identification in the preceding sections, it is evident that unsuccessful re-identification can be connected to the proliferation of duplicates. When an applicant’s data already exists in the database but is not correctly re-identified, for example, due to one of the forms of friction, the possibility of generating a new, duplicate record is real. The excerpts presented in this section will explore how duplicate records can arise within the IND’s operations and give insights into the subsequent work needed to rectify them.
The following interview excerpt underscores how certain departments within the IND are particularly susceptible to generating duplicate identity records, often attributed to factors such as the high volume of applications they handle and disparities in knowledge and experience with the employed search tools:
Yes, specific departments within the IND have that [problem of creating duplicates], which can indeed create a duplicate applicant more often. For example, counter staff can do that. Due to lack of, well, yes, just having less experience with the system. At least with search [functionalities]. Of course, they have to work quickly because they have the applicant in front of them, so to speak. So maybe there is a bit more time pressure. And besides, my department, which is more trained in searching… we may generally be searching a little better in the system. […] We also have the postal department, which is called DRV, digital registration and preparation. And, of course, they have many more applicants [to process] daily, so there is a good chance that they will create a duplicate applicant. But also my department, even though we are very trained in this. We are also still creating duplicate applicants. More than we’d like. (Interview with IND staff member, January 29, 2021)
The interviewee highlights that even in their department, where they possess a solid grasp of the tools, the creation of duplicate records persists. This observation highlights the multitude of factors that may contribute to the generation of duplicates, even among knowledgeable staff. Discussing this matter, another interviewee added that instances of duplicates could also arise when a migration chain partner has previously established a record for an applicant. However, this record does not get properly re-identified:
We [the IND] also have contact with migration chain partners such as the Vreemdeling Politie [national police], the Koninklijke Marechaussee [national gendarmerie] and the like. They can also create applicants themselves. Buitenlandse Zaken [foreign affairs] can also create applicants. And it often happens that sometimes an applicant has already been created by the Vreemdelingen Politie, and then [the applicant] comes to our counter, that [the data] is created again and that it has been created again in a different way. (Interview with IND staff member, November 2, 2020)
In this scenario, an applicant might not have been successfully re-identified from the record of a migration chain partner due to minor disparities in the data. This, in turn, can result in the creation of a separate and distinct record. In that same interview, the interviewee also cited an issue arising when a considerable amount of time has lapsed between applications from the same individuals. They presented a scenario where IND staff processing the application fail to locate the applicant’s old record, subsequently generating a new one. The interviewee gave two examples of how such duplicate records could be identified. One way is during the final stage of the applicant evaluation process, where a higher-up in the organization conducts extra checks before making a final decision (such as granting a residency permit). Another way is if a new document related to the application arrives, which then requires re-identification. In either case, two records are identified as possibly referring to the same individual and will need to be resolved (the “deduplication process”). Here is how they described the process:
So there is a moment someone creates an applicant. And then a new document arrives. And the person after that could be a year later, five years later, he’s looking and actually finding nothing. But the applicant still comes first. And if he then creates it again, he often does not immediately realize it himself, but I get that back or from the decision process from the applicant that occurs twice. Or someone else also receives a document that then comes in and starts looking for the applicant, only to find out that it occurs twice. Then we have a deduplication process that then turns the two applicants into one applicant again. (Interview with IND staff member, November 10, 2020)
To put it concisely, when identifying duplicate records, as indicated in the interview quote, they are flagged and processed through a “deduplication request.” The “Titles and Identity” (T&I) department processes these requests, taking the necessary steps to take the records in question. Deduplication requests can come from various sources, including IND departments and migration chain partners, with requestors completing a designated form for submission to the T&I department. The form outlines the specific data elements that prompted the requestor to identify duplicates and explain the reasons behind the deduplication request. One interviewee from the T&I department shed light on the evolution of this “deduplication request memo” (ontdubbelverzoek memo in Dutch):
Internally, within the IND, such a deduplication memo must be sent. And for those requests, we have a kind of standard analysis. So basically an analysis based on system facts. For example, what comes in it is: we want to know if there is an identity difference between the applicant. Because we’ve actually been working with that since — let’s see — October 2019, when we also focused on identity differences. We call this “identiteitsvraagstukken” [identity question/problem] internally. That needs to be taken care of, and that was never done before. Until we got a case with a difference that we were aware of, hey, if we actually deduplicate files, we choose to make specific identity leading while this is not correct. Because my department just doesn’t have that authority. And that authority is laid down in policy documents, among other things. (Interview with IND staff member, January 29, 2021)
The interviewee explained that the protocols for deduplicating records lacked a standardized approach. However, as the complexities of the deduplication process became more apparent, efforts were made to streamline and standardize the process. The interviewee emphasized the need for such standardization, especially after instances of incorrect decisions. In situations involving two duplicate entities, a decision is required to determine which of them should be designated as the “leading” identity data based on evidence from the systems (“system facts,” further elaborated below).
The following excerpt from another interview underscores the significant weight attached to the decision-making process of deduplicating data records. Within the organization, this process is sometimes metaphorically likened to designating a “survivor” and a “loser” record. Essentially, the “loser” data record is rendered inactive, a potentially impactful action that could lead to real-world complications for the affected individual if carried out inaccurately, given the interconnectedness of documents associated with these records. The interviewee highlights the challenges of losing document and case data traces during deduplication, highlighting the necessity for traceability in the decision-making process within the system for future procedures:
[…] you choose who… we call it “the survivor.” You choose who will be “the survivor” and “the loser.” It sounds very harsh, but that’s how we choose who will be the survivor. And all data from the survivor remains leading. And you can possibly still find somewhere — if you search very well — some personal details, data of when he was born and the like. But certain data and file documents. You really can’t do anymore; unless it is really stated in the file document under which applicant it was. But other than that, you really can’t figure it out anymore. Cases only if file documents are linked to them and that file document contains a case number. But otherwise you really wouldn’t know anymore. There is nowhere that a copy is stored of this was the situation. None of that is there. That is also the reason why people fill out that memo. So that you at least — should it go wrong — that you notice a little, that you can figure out a little. (Interview with IND staff member, November 2, 2020)
To ensure traceability and consistency in the decision-making process within the deduplication, as highlighted by the interviewee, the “Titles and Identity” (T&I) department utilizes a template to gather information from the IND and other relevant systems upon which the decision will be based. This template outlines the various types of “evidence” that IND personnel can gather to make a decision. These accumulated pieces of evidence are meticulously documented within the same memo. Upon analyzing the template and the deduplication process, I found a classification of evidence into two distinct categories, termed “weak evidence” and “strong evidence,” as denoted in the template itself. Weak evidence encompasses elements such as matching addresses, which can be considered a weak indicator due to potential outdatedness or shared addresses among certain groups like asylum seekers residing in the same housing center. Conversely, strong evidence comprises instances where two records possess identical identification documents, a robust indicator owing to the uniqueness of such documents and their clear association with an individual. While I cannot disclose all the specific types of evidence, this categorization highlights a specific ordering of data types, where certain types hold more significance in determining whether the records pertain to the same individual.
It is important to note that some strong evidence can also originate from sources outside the IND, including migration chain partners and other international information systems. An illustrative case is the justification for deduplication when the National Police finds duplicate records by establishing a connection between data from Eurodac or EU-VIS. In such cases, they may leverage data from prominent European Union information systems to establish a link between seemingly separate records within the IND’s database. In this case, even international systems can take priority in the re-identification process. These scenarios of duplicate resolution demonstrate that even on a local level, resolving questions about personal identity data can involve the engagement of international systems.
Based on my analysis, the IND’s deduplication process can be conceptualized as having four stages. In the first stage (1), multiple records potentially referring to the same individual are identified. These records can be discovered through various means, encompassing internal IND and external re-identification procedures or even through an automated process, which will be elaborated on shortly. Subsequently, in the second stage (2), relevant parties are promptly alerted to the existence of these duplicate records through the deduplication memo. In the third stage (3), the T&I department’s staff gathers evidence by investigating the records in question. They use a systematic approach to determine whether the identified duplicates belong to the same individual. To assist in this process, they use a structured form that outlines different categories of evidence that can support their decision-making. Upon the collection and evaluation of evidence, the fourth and final stage (4) involves the actual execution of the deduplication process within the system. In this step, the records will be processed, documents or data will be updated, and one of the identified duplicate records will be marked inactive. Additionally, these steps are logged in the memo, documenting the entire deduplication procedure and the decision-making process, offering transparency and traceability to subsequent procedures. Figure 5.5 visually presents these different stages in the deduplication process.
Figure 5.5: The author’s conceptualization of the IND’s deduplication process.
The process of deduplication resonates with the insights articulated by Loukissas in his exploration of the idea that “all data are local,” suggesting that the act of “normalizing” duplicates offers a lens through which to examine the diversity inherent in data infrastructures (Loukissas 2019). Echoing Loukissas’ insights, we can consider IND’s deduplication process as a lens demonstrating the heterogeneity of re-identification practices. The emergence of duplicate records within the IND’s database is a result of various factors, ranging from the pressures of workload in specific departments to the complexities of handling diverse forms of information, such as handwritten documents or historical data. Moreover, discrepancies in the practices of migration chain partners can lead to the creation of duplicates when their registrations diverge and subsequently fail to re-identify the same individuals. Examining the process of resolving duplicates not only elucidates the operational intricacies within the deduplication process but also offers insights into the challenges posed by heterogeneous re-identification practices.
Additionally, the deduplication process exposes the changing bureaucratic dynamics associated with re-identification practices. Insights gleaned from the interviews suggest that there may have been a time when IND personnel wielded greater bureaucratic discretion in resolving duplicates, including deciding which record to render inactive. However, this discretionary approach may have resulted in inaccurate decisions. Consequently, the introduction of the deduplication request and memo suggests the shift towards a more standardized approach, complete with clearly defined weak and strong evidence criteria. This shift signifies the organization’s endeavor to streamline the deduplication process, minimize the reliance on discretion, enhance transparency and traceability across the entire process, and ensure more consistent and equitable outcomes. Furthermore, this shift suggests a potential redistribution of roles within the organization, particularly regarding the responsibility for thorough applicant re-identification. In some instances, such as due to challenges like time constraints, staff may not have the opportunity to conduct thorough re-identification of applicants, which can result in the creation of duplicate records. Consequently, the department responsible for deduplication has expanded tasks of correcting wrongly re-identified applicants.
While we have delved into the manual identification of duplicates, an unexplored aspect within the deduplication process is an extension of the initial stage: the automated identification of potential duplicate records within the IND’s database. This automated process entails leveraging the capabilities of the ELISE data matching engine to identify duplicate entries based on specified criteria proactively before they escalate into more complex issues. In the following section, we will delve into the mechanics of automated deduplication, providing a lens into the broader complexities inherent in defining identity for re-identification.
5.6.2 Automated duplicate detection and the challenges of defining duplicates in re-identification
The prior interview excerpts have revealed recurring causes for duplicate records being introduced into the INDiGO system. Factors such as time constraints, limited familiarity with the search tools, inadequate training, knowledge gaps, and system integration with external organizations contribute to the creation of duplicates. Multiple records for the same individual pose a risk for the IND to make erroneous decisions, and detecting these duplicates early is therefore considered crucial for the IND (Fieldnotes 2020-07-06). Consequently, the data matching system is employed not only for applicant searches but also to identify potential duplicates using distinct criteria tailored for this purpose.
Upon reviewing available documentation, I uncovered two distinct approaches that could have been adopted for automated duplicate detection within the IND’s re-identification process.41 The first approach involves implementing automated checks during the initial registration of applicants. The second entails periodic automated scans of the entire database to identify potential duplicates. Notably, the IND chose to forego the first option, which would involve automatic checks while creating applicant records. Instead, the organization has opted for the second approach, relying on automated database queries performed at intervals to detect potential duplicates. In practice, this service systematically compares recently created applicant records and all pre-existing database entries. This process aims to identify potential duplicates between a newly generated record and an older one. The IND has distinct criteria for calculating match scores within the ELISE data matching system for this automated process, which differ from the standard criteria used for regular applicant searches. If the computed match score between two records surpasses a predetermined threshold, both records are flagged as potential duplicates, warranting further investigation.
During fieldwork, I had the opportunity to gain further insights into the configuration of the data matching system designed for identifying potential duplicates. These insights were acquired through discussions with the staff of the WCC regarding the development of a new version of the duplicate resolution system (Fieldnotes 2020-07-06). At that time, the previous version encountered performance issues, prompting the development of an updated version to be rolled out. In meetings with WCC staff, I was privy to the considerations and deliberations surrounding configuring data-matching rules for duplicate detection. Although I cannot disclose all the rules, I can share some general principles. Notably, matching criteria included factors such as shared surnames, nationalities, and birth years (with less emphasis on matching months and days of birth). For instance, I was given an example of twins with different names but the same initials and birthdates, which could trigger a false positive in the duplicate detection mechanism if the rules were not carefully set up. This illustrates the complexity of developing matching criteria that are both sensitive enough to identify potential duplicates but also specific enough to avoid incorrect identifications.
What stood out in these discussions was the dual consideration of custom-made solutions versus standardized software. Specifically, the company engaged in developing the deduplication tool was weighing the merits of creating a solution tailored precisely to the IND’s needs versus a more standardized solution that could potentially be deployed for other customers in the future. A member of the project team outlined the contrasting features of these alternatives. On the one hand, a standardized solution would offer a consistent approach to detecting duplicates, making it easier to implement across various customers of the company. However, it might be less adaptable to the specific organizational contexts in which it is deployed. On the other hand, a highly customizable solution could be crafted, albeit requiring significant tailoring to adapt to individual customers’ unique contexts and deduplication requirements. Ultimately, the custom-made solution was deemed the most fitting choice. A concern that was repeatedly raised was the difficulty in creating clear and comprehensive definitions for identity and duplicate records, consistently portrayed as deeply entwined with the specific organizational context.
Delving into these automated mechanisms not only uncovers the complexities of identifying and managing duplicates but also underscores their entanglement with street-level, screen-level, and system-level bureaucracies. Duplicates often emerge due to failed re-identification, which can occur at any of these three levels. Moreover, the resolution of these duplicates can occur at any of these three levels. Offline deduplication, characterized by periodic reviews of potential duplicate records, aligns more with system-level operations. In contrast, online deduplication operates in real-time, affecting street and screen-level bureaucrats immediately during their daily workflow. Furthermore, the choice between a standardized and customized deduplication approach underscores how identity and duplicate record definitions relate to organizational contexts. Chapter 6 will delve deeper into the intricacies of constructing data matching systems to work across organizations. However, before we proceed, the upcoming section will introduce an analytical tool designed to interpret the diversity of re-identification practices discussed thus far.
5.7 An interpretative framework for re-identification scenarios based on search input and results
This section sets out to synthesize the chapter’s findings to address the research question that has guided this chapter’s investigation: “How do organizations that collect information about people-on-the-move search and match for identity data in their systems? How is data about people-on-the-move matched and linked across different agencies and organizations?” As we navigated the multiple facets of re-identification at the IND and the migration chain, these questions can now be answered by synthesizing the findings into a typology of re-identification scenarios. As such, this analysis serves a dual purpose: it unveils the operational intricacies of data matching in migration management’s bureaucratic procedures while delving into the challenges, friction, and complexities that weave through these procedures.
In light of the chapter’s findings, it becomes evident that re-identification within IND can take different forms. On the one hand, re-identification is characterized by the diverse information available to staff during the process: more or less skills can be required to interpret information to be inputted in the system. On the other, the precision requirements for successful re-identification differ significantly: more or less skills can be required to interpret search results. Building on these observations, I propose an analytical framework for interpreting re-identification that categorizes practices based on the demands of interpreting search inputs and results. This classification yields four distinct combinations, visually depicted as a matrix in Figure 5.6. This interpretative framework serves as a tool for unraveling the intricacies of re-identification within street-level, screen-level, and system-level bureaucracies, enabling a clearer understanding of the diverse impacts of data frictions and the ensuing costs that arise in the event of re-identification failures.
Figure 5.6: This matrix shows the four combinations arising from differences in the need for interpreting the input with the need for interpreting the results in re-identification.
In the upper left quadrant of the matrix, re-identification scenarios are depicted where the need for scrutinizing both input and results is relatively low. This often pertains to routine tasks involving unambiguous data like identification numbers, frequently conducted at service counters where direct interactions with applicants occur. An illustrative instance is when staff need only the v-number to access existing applicant data, leading to straightforward search results to retrieve the applicant’s data. This quadrant also includes instances of automated search and matching processes, such as the automated exchange of residency data. In these cases, the search query is predefined, meaning it’s set in advance without the need for interpretation by IND staff, as the data originates from another source. Moreover, the system doesn’t interpret the results like a human would; instead, it simply selects the outcome with the highest match score from the available matches.
The upper right quadrant pertains to re-identification scenarios wherein the input may have a slightly higher level of ambiguity, yet the necessity for reviewing output remains relatively modest. This quadrant encompasses scenarios involving tasks like handling phone calls or processing written documents; it includes scenarios where the applicant being searched for is typically already present within the system, leading to fewer searches and a decreased demand for comprehensive familiarity with search functionalities. In this context, the primary challenge for re-identification originates from the necessity to interpret the input. For instance, this might involve deciphering an applicant’s v-number provided over the phone or transcribing data from a written form into the search query. This process can introduce errors that affect the search results, for instance, due to audible misunderstandings or typographical mistakes.
The lower-left quadrant focuses on re-identification scenarios characterized by a diminished requirement for reviewing input, as it has typically pre-established, but a heightened necessity for scrutinizing results due to the careful interpretation they demand. This quadrant encompasses instances like the processing of automated deduplication matches and the tasks by what is termed as “decision makers” within the organization. In automated deduplication, the input is also predefined, but considerable effort is necessary to verify potential duplicates meticulously. The notion of decision makers pertains to individuals within the organization, often superiors, who conduct final assessments before arriving at decisions concerning applicants. In both these scenarios, a rigorous validation process is essential to ascertain whether an individual is already present in the system, leading to a higher volume of searches. Consequently, this demands a thorough familiarity with search functionalities and customized search methodologies.
Finally, the lower-right quadrant encompasses re-identification scenarios characterized by ambiguous input and a need to examine search results thoroughly. Scenarios falling within this quadrant are exemplified by the initial registration and commencement of a procedure for an applicant, particularly when dealing with processing written application forms. Here, staff may encounter the challenge of deciphering potentially unclear and error-ridden forms. Simultaneously, thorough inquiry is required to ascertain whether an individual is not already enlisted within the IND or migration chain. This is necessary to ensure that an applicant has, for instance, not been previously registered under a different name before marriage. Consequently, as revealed by the findings, such cases demand a familiarity with the search and match tools and often prompt staff to devise their own search strategies.
The matrix consisting of four distinct combinations functions as an interpretative framework, enabling us to comprehend the nuances of various re-identification scenarios within the entanglement of street-, screen-, and system-level bureaucracies and how different scenarios interact with and respond to different forms of data friction. The framework categorizes these differences as contingent upon specific levels of input and output review requisites. In the upper two dimensions of the matrix, there is a higher certainty that the applicant is already within the system. This leads to scenarios characterized by fewer searches, less reliance on intricate search features, and potential friction primarily related to processing search results. In contrast, the lower two dimensions signify greater uncertainty about the applicant’s presence in the system. More searches are needed in these situations, a comprehensive understanding of search features is crucial, and customizing search parameters becomes essential. The potential forms of friction in re-identification are likely more influenced by the complexities at the input stage and by differences in re-identification practices.
Re-identification scenarios in bureaucratic settings exhibit a complex interplay that extends across street-level, screen-level, and system-level operations. Street- and screen-level bureaucrats often initiate the data entry process, gathering and inputting information into the system. This data then undergoes subsequent processing where applicants are re-identified for verifying, updating, and correcting applicant details. Throughout this process, these bureaucrats grapple with data friction stemming from disparities between the information provided by applicants and the data present in official records. Their ability to achieve accurate re-identification relies on interacting with system interfaces that shape the process at various stages – from entering search queries to interpreting the ranked results. Meanwhile, system-level decisions revolving around matching algorithms, criteria, and technical configurations wield influence over the outcomes of re-identification efforts.
5.8 Conclusion
This chapter commenced by highlighting the significance of re-identification processes, even in high-stake cases like that of the Boston bomber, where specialized technologies are employed by authorities to navigate ambiguous re-identification outcomes, as evidenced by the ambiguity surrounding the perpetrator’s transliterated name. Notably, participants in the study also highlighted security considerations, such as the role of duplicate record detection in identifying individuals with malicious intent. However, a more comprehensive exploration of the connection between data matching and security will be addressed in the subsequent chapter. Shifting away from such high-stakes contexts, the investigation delved into re-identification processes as routine bureaucratic practices supported by technological tools aimed at minimizing uncertainties. Through a comprehensive exploration of the Netherlands’ Immigration and Naturalization Service (IND) and its interconnections with the Dutch migration chain, the research set out to examine the multifaceted practices of re-identifying applicants.
The inquiry began by examining the IND’s designed infrastructure for applicant re-identification, including the tools for searching and matching applicants’ identity data. Next, this designed infrastructure was compared through its practical implementation and use, enabling us to unravel the challenges inherent in the IND’s identification processes. The analysis identified three prominent forms of data friction that may hinder applicant re-identification: friction between standardized identification and the differences in institutional practices, friction from variations in the precision and accuracy of identity data during its transformation across different mediums and use in formulating search queries, and friction arising from the opaque calculation of match results and the need for thorough interpretation and fine-tuning of search results. These forms of friction, in turn, prompted a closer examination of the costs arising from failed re-identification, as exemplified by the existence of duplicate records and the labor-intensive process of deduplication.
The findings enrich our theoretical understanding of re-identification processes in three dimensions. Firstly, re-identification is more than the technical mechanisms of matching data; it encompasses complex negotiations between diverse identification practices. It involves the IND acting as a mediator in the complex web of bureaucratic operations, aligning multifaceted identification practices for successful re-identification. Secondly, the data matching system’s standardized design interacts dynamically with individual user needs during practical application. This interaction underscores the complexity of translating standardized designs into effective practice, particularly within the intricate context of the IND’s operations. These findings emphasize that successful re-identification requires a confluence of designed features, user adaptations, and real-world intricacies. Lastly, the expertise needed for successful re-identification is through a synergy of human and technological data matching expertise. The interviewees’ comprehension of the data matching system was partial, as computer systems employ autonomous functionalities beyond their explicit awareness. Moreover, the specific match criteria often remain opaque. This distribution of knowledge signifies a division of labor, where human operators rely on computer systems to enhance their re-identification efforts. However, successful re-identification also rests on the tricks of the trade of human operators in refining, comprehending, and critically evaluating search results.
Examining the costs of failed re-identification through the existence of duplicate records and the labor-intensive process of deduplication contributed two insights into re-identification. Firstly, delving into the process of resolving duplicates not only unveiled the operational intricacies of deduplication but also offered a lens to understand the evolving dynamics of bureaucratic re-identification practices. Interviews indicated that there may have been a period when IND personnel exercised greater bureaucratic discretion in resolving duplicates, potentially leading to inaccurate decisions. Consequently, the shift towards a standardized approach, marked by pre-defined evidence criteria, reflects the organization’s pursuit of streamlining deduplication, reducing discretionary elements, enhancing transparency and traceability, and ensuring more consistent outcomes. Secondly, the automated duplicate detection process, driven by the data matching engine, presents a proactive solution to enduring re-identification challenges. However, delving into these automated mechanisms not only reveals the complexities of duplicate identification but also underscores the broader intricacies inherent in defining identity. The struggle to establish a universal deduplication method underscores that such definitions are inherently tied to an organization’s context, defying easy application to other organizations.
These findings were synthesized to create an interpretative framework that conceptualizes re-identification practices according to the demands of interpreting search inputs and results. This resultant matrix of diverse re-identification scenarios effectively serves as a bridge to address the gaps identified in the literature review. The literature review unveiled two overarching themes regarding the conceptualization of re-identification as a bureaucratic practice. We recognized re-identification as a substantial yet relatively unexplored aspect of bureaucratic interactions with applicants, particularly as these interactions shift from traditional in-person settings toward more digitally-mediated and automated processes, characterized in the literature as a transition from street-level to screen-level and system-level bureaucracies (Bovens and Zouridis 2002). The matrix of re-identification scenarios illustrates the multiplicity of practices, showcasing the diverse ways data matching tools shape the processes of re-identifying individuals within bureaucratic contexts. These scenarios encompass various situations, ranging from direct applicant interactions to staff managing phone calls and handling application forms sent via post.
Furthermore, in the literature discussing materialist and performative viewpoints of identification and the challenges arising from data uncertainties and data friction, a noticeable knowledge gap exists concerning how practitioners effectively manage the intricacies of uncertain personal identity data during the identification processes. This gap prompted exploring the effects of technologies designed to address data uncertainties on bureaucratic re-identification practices, potentially enhancing and limiting their efficacy. The matrix visually represents the varying input and output reviews required across distinct re-identification scenarios. Through its typology, the matrix categorizes uncertainties and ambiguities present not only in search inputs but also in search results. Consequently, the findings emphasize that the approach practitioners adopt in navigating uncertainties within personal identity data during identification encounters is context-dependent and shaped by the tools and mechanisms of data matching.
This chapter’s findings also address the dissertation’s central research question: “How are practices and technologies for matching identity data in migration management and border control shaping and shaped by transnational commercialized security infrastructures?” Through examining applicant re-identification practices within the Netherlands’ Immigration and Naturalization Service (IND) and the larger migration chain context, this chapter demonstrated the interconnection between a government migration agency’s re-identification practices and commercial data matching systems, exemplified by the ELISE software. Furthermore, the examination of the deduplication process reveals a crucial link with transnational systems, as the IND and migration chain partners leverage data from prominent European Union information systems to establish connections between seemingly disparate records within their databases. The findings underscore that re-identification processes and technologies are not isolated; they are entwined with wider commercialized security infrastructures.
Several questions remain unresolved regarding the interplay of matching identity data within migration management and border control and their interactions with transnational commercialized security infrastructures. The integration of commercial and proprietary tools, crafted by a private entity, for data matching, suggests an influential shift in the IND’s re-identification knowledge. This integration intertwines the core of re-identification knowledge with a specialized system, resulting in a scenario where significant expertise resides within this proprietary system. However, the recent upgrade of the IND’s deduplication tools illustrates the tension between crafting generic versus context-specific data matching systems. Such tensions raise questions about the potential role of the generic design of ELISE and software vendors’ strategies for “generification” (Pollock and Williams 2009). Regrettably, the impact of software vendors and their broader market strategies remains largely unexplored in current research. Thus, the subsequent chapter will illuminate the software’s evolution, shedding light on how knowledge and technology related to matching identity data traverse diverse organizational boundaries.
References
About, Ilsen, James Brown, and Gayle Lonergan, eds. 2013a. Identification and Registration Practices in Transnational Perspective. St Antony’s Series. London: Palgrave Macmillan. https://doi.org/10.1057/9781137367310.
Basis Technology. 2021. “Strengthening U.S. Borders with Intelligent Name Matching.” Rosette Text Analytics: Case Studies. https://web.archive.org/web/20211105135209/https://www.rosette.com/case-studies/us-customs-border-protection/.
Bates, Jo. 2017. “The Politics of Data Friction.” Journal of Documentation 74 (2): 412–29. https://doi.org/10.1108/JD-05-2017-0080.
Batini, Carlo, and Monica Scannapieco. 2016. “Object Identification.” In Data and Information Quality: Dimensions, Principles and Techniques, edited by Carlo Batini and Monica Scannapieco, 177–215. Data-Centric Systems and Applications. Cham: Springer International Publishing. https://doi.org/10.1007/978-3-319-24106-7_8.
Bellanova, Rocco, and Georgios Glouftsios. 2022. “Controlling the Schengen Information System (SIS II): The Infrastructural Politics of Fragility and Maintenance.” Geopolitics 27 (1): 160–84. https://doi.org/10.1080/14650045.2020.1830765.
Bergsma, Bouke. 2013. “Systeem IND duurder en trager ingevoerd.” Algemeen Nederlands Persbureau ANP, November. https://web.archive.org/web/20201013120039/https://www.nu.nl/binnenland/3628805/systeem-ind-duurder-en-trager-ingevoerd.html.
Bovens, Mark, and Stavros Zouridis. 2002. “From Street-Level to System-Level Bureaucracies: How Information and Communication Technology Is Transforming Administrative Discretion and Constitutional Control.” Public Administration Review 62 (2): 174–84. https://doi.org/10.1111/0033-3352.00168.
Bowker, Geoffrey C., and Susan Leigh Star. 1999. Sorting Things Out: Classification and Its Consequences. Inside Technology. Cambridge, Mass.: The MIT press.
Breckenridge, Keith, and Simon Szreter, eds. 2012. Registration and Recognition: Documenting the Person in World History. Oxford: Oxford University Press for the British Academy.
Buffat, Aurélien. 2015. “Street-Level Bureaucracy and E-Government.” Public Management Review 17 (1): 149–61. https://doi.org/10.1080/14719037.2013.771699.
Busch, Peter André, and Helle Zinner Henriksen. 2018. “Digital Discretion: A Systematic Literature Review of ICT and Street-Level Discretion.” Information Polity 23 (1): 3–28. https://doi.org/10.3233/IP-170050.
Caplan, Jane, and John Torpey, eds. 2001. Documenting Individual Identity: The Development of State Practices in the Modern World. Princeton, N.J.; Oxford: Princeton University Press.
Christen, Peter. 2012. Data Matching: Concepts and Techniques for Record Linkage, Entity Resolution, and Duplicate Detection. Data-Centric Systems and Applications. Berlin; New York: Springer. https://doi.org/10.1007/978-3-642-31164-2.
Cole, Simon A. 2001. Suspect Identities: A History of Fingerprinting and Criminal Identification. Cambridge, Mass.; London, Eng.: Harvard University Press.
Collins, Stephanie Baker. 2016. “The Space in the Rules: Bureaucratic Discretion in the Administration of Ontario Works.” Social Policy and Society 15 (2): 221–35. https://doi.org/10.1017/S1474746415000251.
Cresswell, Tim. 2010. “Towards a Politics of Mobility.” Environment and Planning D: Society and Space 28 (1): 17–31. https://doi.org/10.1068/d11407.
ECA, European Court of Auditors. 2020. EU Information Systems Supporting Border Control: A Strong Tool, but More Focus Needed on Timely and Complete Data. Special Report No 20, 2019. Luxembourg: Publications Office of the European Union. https://data.europa.eu/doi/10.2865/83092.
Edwards, Paul N. 2010. A Vast Machine: Computer Models, Climate Data, and the Politics of Global Warming. Cambridge, Mass.; London, Eng: The MIT Press.
European Union Agency for Fundamental Rights. 2018. Under Watchful Eyes: Biometrics, EU IT Systems and Fundamental Rights. Luxembourg: Publications Office of the European Union. https://data.europa.eu/doi/10.2811/136698.
Fors-Owczynik, Karolina La, and Irma van der Ploeg. 2015. “Migrants at/as Risk: Identity Verification and Risk-Assessment Technologies in the Netherlands.” In Digitizing Identities, edited by Irma van der Ploeg and Jason Pridmore, 261–81. Routledge Studies in Science, Technology and Society 30. New York; London: Routledge.
Friese, Susanne. 2014. Qualitative Data Analysis with ATLAS.Ti. Second. London: SAGE Publications Ltd.
Gargiulo, Enrico. 2017. “Monitoring or Selecting? Security in Italy Between Surveillance, Identification and Categorisation.” In Rethinking Surveillance and Control: Beyond the "Security Versus Privacy" Debate, edited by Elisa Orrù, Maria Grazia Porcedda, and Sebastian Weydner-Volkmann, 195–215. Baden-Baden: Nomos.
Gitelman, Lisa, ed. 2013. “Raw Data” Is an Oxymoron. Infrastructures. Cambridge, Mass.: The MIT press.
Grijpink, Jan. 1997. “Chain-Computerisation for Interorganisational Public Policy Implementation.” Information Infrastructure and Policy 6 (2): 81–93. https://content.iospress.com/articles/information-infrastructure-and-policy/iip082.
ICTU. 2015. “Informatievoorziening Vreemdelingenketen.” ICTU Publicaties. https://web.archive.org/web/20190525093722/https://www.ictu.nl/publicaties/informatievoorziening-vreemdelingenketen.
Keulen, Maurice van. 2012. “Managing Uncertainty: The Road Towards Better Data Interoperability.” IT - Information Technology 54 (3): 138–46. https://doi.org/10.1524/itit.2012.0674.
KPMG IT Advisory. 2011. “Audit INDiGO: ‘Willen, Kunnen En Doen’.” Audit. The Hague, The Netherlands.
Landsbergen, David. 2004. “Screen Level Bureaucracy: Databases as Public Records.” Government Information Quarterly 21 (1): 24–50. https://doi.org/10.1016/j.giq.2003.12.009.
Leese, Matthias. 2022. “Fixing State Vision: Interoperability, Biometrics, and Identity Management in the EU.” Geopolitics 27 (1): 113–33. https://doi.org/10.1080/14650045.2020.1830764.
Lipsky, Michael. 2010. Street-Level Bureaucracy: Dilemmas of the Individual in Public Services. 30th anniversary expanded. New York: Russell Sage Foundation.
Loukissas, Yanni Alexander. 2019. All Data Are Local: Thinking Critically in a Data-Driven Society. Cambridge, Mass.: The MIT Press.
Lyon, David. 2009. Identifying Citizens: ID Cards as Surveillance. Cambridge, UK; Malden, MA: Polity.
Ministerie van Justitie en Veiligheid. 2023. “Thema’s En Architectuurprincipes - MIRA-Online.” http://web.archive.org/web/20230802095011/https://www.miraonline.nl/index.php/Thema%27s.
Ministerie van Justitie en Veiligheid. 2022. “Protocol identificatie en labeling: gestandaardiseerde werkwijze voor de unieke identificatie en registratie in de migratieketen.” Richtlijn Versie 12.1. Ministerie van Algemene Zaken. http://web.archive.org/web/20230906100658/https://open.overheid.nl/documenten/ronl-041514e0-ffef-448f-acfe-626fd7c8160e/pdf.
Oosterbaan, Teun. 2012. “Architectuur Als Agenda: Een Theoretische En Empirische Analyse van de Rol van Frames Bij Architectuurontwikkeling Voor Keteninformatisering.” PhD thesis, Erasmus University Rotterdam. http://hdl.handle.net/1765/31677.
Pallitro, Robert, and Josiah Heyman. 2008. “Theorizing Cross-Border Mobility: Surveillance, Security and Identity.” Surveillance & Society 5 (3). https://doi.org/10.24908/ss.v5i3.3426.
Pelizza, Annalisa. 2016b. “Disciplining Change, Displacing Frictions: Two Structural Dimensions of Digital Circulation Across Land Registry Database Integration.” Tecnoscienza. Italian Journal of Science & Technology Studies 7 (2): 35–60.
Pelizza, Annalisa. 2021. “Identification as Translation: The Art of Choosing the Right Spokespersons at the Securitized Border.” Social Studies of Science 51 (4): 487–511. https://doi.org/10.1177/0306312720983932.
Pelizza, Annalisa, and Wouter Van Rossem. 2023. “Scripts of Alterity: Mapping Assumptions and Limitations of the Border Security Apparatus Through Classification Schemas.” Science, Technology, & Human Values 0 (0): 1–33. https://doi.org/10.1177/01622439231195955.
Pollock, Neil, and Robin Williams. 2009. Software and Organisations: The Biography of the Enterprise-Wide System or How SAP Conquered the World. Routledge Studies in Technology, Work and Organisations 5. London; New York: Routledge.
Pollozek, Silvan, and Jan Hendrik Passoth. 2019. “Infrastructuring European Migration and Border Control: The Logistics of Registration and Identification at Moria Hotspot.” Environment and Planning D: Society and Space 37 (4): 606–24. https://doi.org/10.1177/0263775819835819.
Salter, Mark B. 2013. “To Make Move and Let Stop: Mobility and the Assemblage of Circulation.” Mobilities 8 (1): 7–19. https://doi.org/10.1080/17450101.2012.747779.
Schmitt, Eric, and Michael S. Schmidt. 2013. “2 U.S. Agencies Added Boston Bomb Suspect to Watch Lists.” The New York Times, April. https://web.archive.org/web/20210513103658/https://www.nytimes.com/2013/04/25/us/tamerlan-tsarnaev-bomb-suspect-was-on-watch-lists.html.
Scott, James C. 1998. Seeing Like a State: How Certain Schemes to Improve the Human Condition Have Failed. New Haven; London: Yale University Press.
Skinner, David. 2018. “Race, Racism and Identification in the Era of Technosecurity.” Science as Culture 29 (1): 77–99. https://doi.org/10.1080/09505431.2018.1523887.
Snellen, Ignace. 2002. “Electronic Governance: Implications for Citizens, Politicians and Public Servants.” International Review of Administrative Sciences 68 (2): 183–98. https://doi.org/10.1177/0020852302682002.
Suchman, Lucy, Karolina Follis, and Jutta Weber. 2017. “Tracking and Targeting: Sociotechnologies of (in)Security.” Science, Technology, & Human Values 42 (6): 983–1002. https://doi.org/10.1177/0162243917731524.
Toet, Diederik. 2009. “Indigo-systeem van IND wint architectuurprijs.” Computable.nl. https://web.archive.org/web/20201013115613/https://www.computable.nl/artikel/nieuws/crm/3174763/2333360/indigosysteem-van-ind-wint-architectuurprijs.html.
Torpey, John C. 2018. The Invention of the Passport: Surveillance, Citizenship and the State. Second. Cambridge Studies in Law and Society. New York, NY; Cambridge, UK: Cambridge University Press. https://doi.org/10.1017/9781108664271.
van der Ploeg, Irma. 1999. “The Illegal Body: ‘Eurodac’ and the Politics of Biometric Identification.” Ethics and Information Technology 1 (4): 295–302. https://doi.org/10.1023/A:1010064613240.
Van Rossem, Wouter, and Annalisa Pelizza. 2022. “The Ontology Explorer: A Method to Make Visible Data Infrastructures for Population Management.” Big Data & Society 9 (1): 1–18. https://doi.org/10.1177/20539517221104087.
Winter, Tom. 2014. “Russia Warned U.S. About Tsarnaev, but Spelling Issue Let Him Escape.” NBC News, March. https://web.archive.org/web/20210330213852if_/https://www.nbcnews.com/storyline/boston-bombing-anniversary/russia-warned-u-s-about-tsarnaev-spelling-issue-let-him-n60836.
Zijderveld, Marianne, Willem Ridderhof, and Marco Brattinga. 2013. “Basis start architectuur architectuur van de vreemdelingenketen: kennis delen, informatie gebruiken, samen doen.” Den Haag: Ministerie van Binnenlandse Zaken. http://web.archive.org/web/20220901074647/https://www.digitaleoverheid.nl/wp-content/uploads/sites/8/2017/01/architectuur-van-de-vreemdelingenketen.pdf.
Tech companies often use the Boston bomber’s misspelt names as an example of why watch list screening systems need their data matching technologies (see also Basis Technology 2021). Businesses can use this scenario in their sales pitch to show how their technology can handle the ambiguity in identifying and connecting individuals’ identities. Despite the risk of perpetuating the securitization of identification, this case is instructive as a practical illustration of the interdependence of various government agencies, border guards, and watchlisting systems in identifying potentially risky travelers.↩︎
The General Data Protection Regulation (GDPR), for instance, states the accuracy principle in Article 5(1)d. According to this principle, the personal data that organizations collect and use must be “accurate and, where necessary, kept up to date,” and “every reasonable step must be taken to ensure that personal data that are inaccurate, having regard to the purposes for which they are processed, are erased or rectified without delay.”↩︎
In technical literature, the term re-identification is also used to describe processes of de-anonymizing data, i.e., revealing personal identities associated with anonymized data. Re-identification of previously anonymized individuals is, in fact, always a possible outcome of data matching processes. As Christen (2012) explains, re-identification is possible because “record pairs classified as matches in a data matching project can contain information that is not available in the individual source databases that were matched” (p. 189). By matching data from different sources, individuals in those databases may be (un)intentionally identified and disclosed even with incomplete identifying information. Consequently, there is an undeniable connection between re-identification as de-anonymization and the practices and technologies described in this chapter.↩︎
In this chapter, the term “applicant” is employed to refer to the individuals that submit formal requests or applications to the government agency. However, the prevalent term used by interview participants from the agency in Dutch is “klant” or “cliënt.” This term would translate to “client,” a person receiving the benefits or services of a government agency. Interestingly, the first term could also be translated to “customer.” I have chosen to utilize “applicants” to refer to these individuals as “applicant” emphasizes the act of making a request or application, whereas “client” emphasizes the recipient of a service or assistance.↩︎
A new architecture called MIRA is being developed for the migration chain. Unlike the current approach of automating data streams between chain partners, this new architecture suggests creating an information platform that offers data services for chain partners to use in achieving their processes, services, and systems (Ministerie van Justitie en Veiligheid 2023).↩︎
The system is based on Oracle’s Siebel system, a multinational computer technology company. While it is interesting to consider how this generic Siebel case management system has influenced the IND’s operations, it is out of scope for this discussion.↩︎
INDiGO documentation refers to this as the “flow” (information management) from the “know” (policy implementation). Technically, this separation is accomplished by adopting a Service-Oriented Architecture (SOA) design, a software architecture strategy that aims to modularize system functions into relatively independent services.↩︎
The architecture of the INDiGO system received praise during its initial development in 2009 (Toet 2009), but the rollout of the new system in subsequent years did face several delays and problems (Bergsma 2013).↩︎
Technically, this was made possible by running the software’s algorithms on data that has been replicated from both INDIS and INDiGO (i.e., regularly copying data from both sources into the ELISE in-memory database). The initial rollout of the INDiGO system started in 2009 and was completed in 2013.↩︎
It is noteworthy that initially, INDiGO utilized ELISE’s fingerprint-matching capabilities to verify whether an applicant was already known, achieved by comparing their fingerprints during their first registration with the entire database. However, this “one-to-many” matching practice is no longer in use. Fingerprint data now exclusively serves to confirm an individual’s identity, particularly during face-to-face interactions with applicants at service counters. A simplified process involving distinct software is employed for this purpose, enabling “one-to-one” fingerprint matching. Although biometrics play a role, this chapter’s primary focus revolves around backstage re-identification and the utilization of non-biometric data. This emphasis corresponds with the identified gap in the existing literature.↩︎
This also influenced by the way INDiGO utilizes the ELISE system. ELISE has the capability to dynamically adjust the behavior and importance of search criteria for specific services, which isn’t the case in the INDiGO implementation. The GUI lacks the feature to configure this for queries, and it seems that the criteria used in data matches are not displayed either. This is likely a result of balancing user-friendliness, configurability, and complexity of implementation.↩︎
INDiGO’s technical design documents refer to these two approaches to finding possible duplicates as online and offline deduplication.↩︎